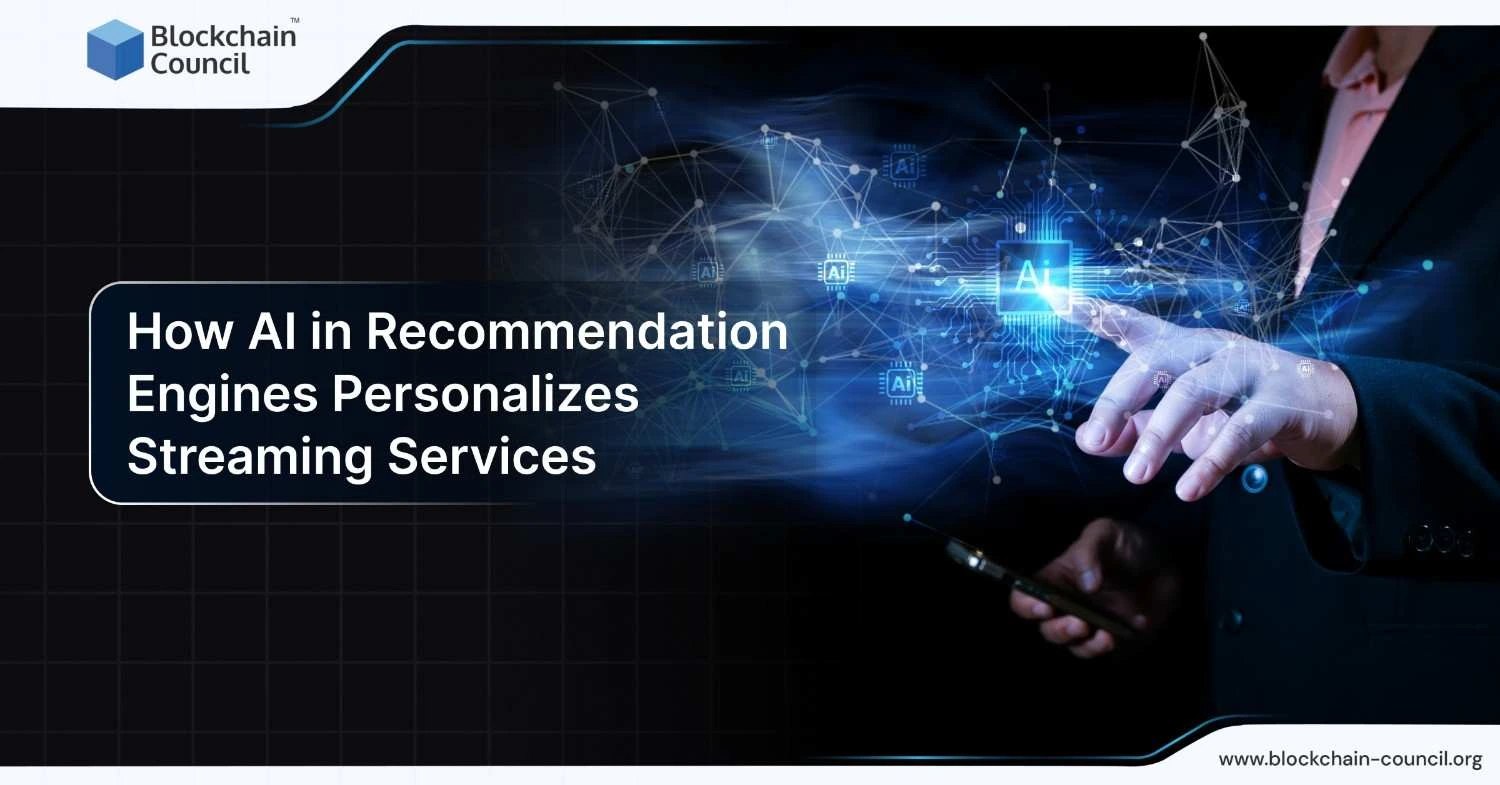
- Blockchain Council
- October 21, 2024
The use of AI in recommendation engines has changed the way streaming platforms personalize content for their users.
What Are AI-Powered Recommendation Engines?
AI-driven recommendation systems are designed to suggest content based on users’ previous actions and preferences. These engines work by using machine learning to handle vast amounts of data gathered from user activity. This includes what people watch, how long they stay on certain content, and even their ratings or reviews. By sorting through all this data, the systems create recommendations that match the viewer’s tastes, keeping them engaged with the platform.
Streaming services like Netflix, Spotify, and YouTube have been using these AI-based systems for many years, but their methods have gotten more advanced over time. They now use a mix of different approaches, such as collaborative filtering, content-based filtering, and even hybrid systems, to provide personalized recommendations. For example, Netflix has stated that over 80% of the content watched on its platform is influenced by its recommendation engine.
Writing precise prompts is essential in AI interactions, especially when building recommendation engines. Earning the Certified Prompt Engineer™ certification helps refine this skill for those involved in personalization technologies.
How Do These Engines Work?
Data Collection and Analysis
AI engines depend a lot on data. They gather details from users’ activities, like what they’ve watched, whether they paused or skipped certain content, and what kind of ratings they gave. Platforms also track other factors, such as how long a user spends watching a particular genre or how often they re-watch content. All this information is used to build profiles for both the users and the content available on the platform.
Collaborative Filtering
One way these systems make suggestions is through collaborative filtering. This method compares users who have similar preferences and suggests content based on what others with similar tastes enjoy. It works especially well when there is a large group of users to analyze because patterns become easier to spot. However, this approach might struggle when dealing with new users who haven’t interacted much with the platform yet.
Content-Based Filtering
Another technique is content-based filtering. Instead of comparing users, it looks at the characteristics of the content itself. For example, it may analyze genres, actors, or specific themes in a movie or show and recommend something similar to what a user has liked before. Spotify, for instance, uses details like tempo, mood, and key in songs to make music recommendations. This helps ensure that users receive suggestions based on both their preferences and the attributes of the content.
Hybrid Systems
Platforms like Netflix and Spotify often use a combination of both collaborative and content-based filtering, known as hybrid systems. By blending these approaches, they can offer users more accurate and personalized content. The system looks at both a user’s past behavior and what others with similar tastes are enjoying, creating a broader range of suggestions.
To truly deepen your understanding of AI and its application in recommendation engines, the Master Artificial Intelligence (AI) Learning Path could be the perfect step toward advancing your role in personalized streaming.
Real-World Examples of AI Personalization
Netflix
Netflix’s recommendation engine plays a central role in how users find new shows or movies. As of 2024, the platform has over 260 million active users. Its AI system doesn’t just suggest what to watch; it adapts to changing preferences over time. For example, Netflix tailors the layout of your homepage and recommendations so that you can find something interesting to watch quickly. This has helped the platform keep more viewers engaged, contributing to its success in the streaming market.
Spotify
Spotify’s “Discover Weekly” and “Daily Mix” playlists are powered by its AI system. By looking at your listening habits, Spotify puts together playlists that feel personal, based on what you’ve chosen in the past. In 2023, Spotify took things a step further by analyzing the actual audio of songs to make even better recommendations. This means the playlists not only reflect what you’ve listened to but also capture the overall mood you’re in.
YouTube
YouTube’s recommendation system is also built around AI. It tracks a user’s viewing history and interactions, such as likes and shares, to suggest videos. YouTube’s AI uses deep learning to understand not only what content you engage with but also the time and device you use to watch it. This allows the platform to offer up videos that feel relevant throughout the day.
Personalization on Other Platforms
Other platforms, like Tubi, are adding AI-driven features too. Tubi’s “Rabbit AI” allows users to search for content through natural language. So, you can ask things like “What are some fun movies for tonight?” and get personalized suggestions instantly. This conversational approach makes finding content easier and more user-friendly.
Hulu, Amazon Prime, and Disney+ also use AI-powered engines. Like Netflix, they combine methods such as collaborative filtering (based on user interactions) with content-based filtering (looking at the content itself). This dual method means that they can cater to individual tastes while also introducing new content that users may not have considered.
AI plays a vital role in building recommendation engines. Earning the Certified Artificial Intelligence (AI) Developer™ credential will better prepare you to design systems that improve personalized streaming experiences.
Contextual and Dynamic Recommendations
Today, recommendation engines have become more advanced, adjusting suggestions based on things like the device being used or the time of day. For instance, what you might see as a suggestion on a Wednesday afternoon may differ from what you see on a Saturday night. This makes recommendations more relevant to the context in which you are viewing content.
Additionally, many streaming services now offer real-time personalization. As users engage with content, the recommendations update instantly. If someone watches comedies during the week but prefers documentaries on weekends, the system can shift the recommendations accordingly.
In the competitive AI space, the Certified Artificial Intelligence (AI) Expert™ title can set you apart and demonstrate your ability to innovate in areas like recommendation engines for streaming services.
Ethical Concerns and Challenges
While AI has certainly made personalization better, it comes with challenges. For one, the amount of data being collected can raise privacy concerns. Users might not always know just how much information is being tracked. There’s also the risk of users getting stuck in “filter bubbles” where they are only shown content that matches their current interests, limiting exposure to other types of content.
Another concern is transparency. Streaming platforms need to make sure that users understand how recommendations are made, giving them control over their content choices.
Final Thoughts
AI recommendation engines have changed how we interact with streaming services. By analyzing user habits and preferences, these systems offer personalized suggestions that keep people engaged. While there are challenges, especially around privacy and content variety, the advantages of these AI systems are clear: they make finding content easier and more relevant.
The Unlimited Learning Subscription (AI) ensures that professionals working with recommendation engines in streaming are always up to date on AI’s role in it.