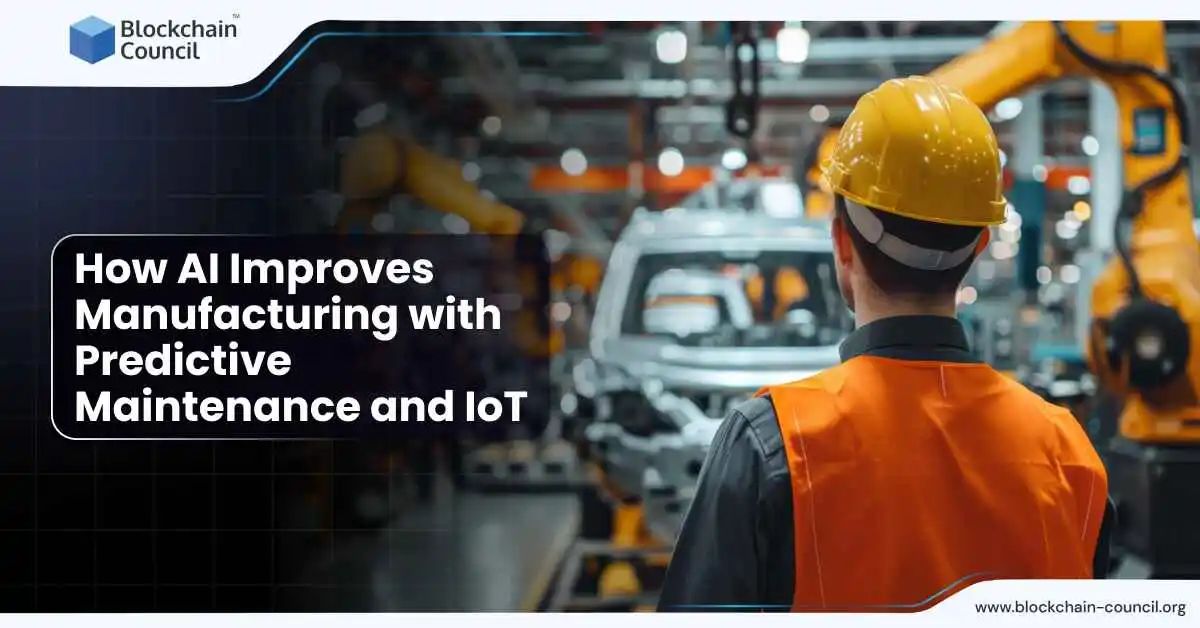
- Blockchain Council
- October 28, 2024
Artificial intelligence (AI) is changing the way manufacturing works, especially with predictive maintenance and IoT (Internet of Things) playing key roles. Industry 4.0 has pushed these changes forward as businesses adopt new technology to remain competitive in the market.
An Overview of AI-Driven Predictive Maintenance
Predictive maintenance is a method where data is used to foresee when machines might fail. This allows manufacturers to service equipment before any serious problems develop. In contrast, traditional methods use set schedules, which can sometimes cause unneeded downtime or surprise breakdowns. AI, through machine learning, evaluates massive datasets gathered from sensors and other IoT tools. This analysis helps spot patterns, detect abnormalities, and predict failures before they happen.
One major advantage of AI in this space is minimizing downtime. With continuous monitoring, AI can pinpoint slight shifts in performance, hinting at a future issue. For instance, Siemens has applied AI in their plants, helping them streamline processes, which has not only boosted their efficiency but also reduced the need for expensive repairs.
How AI and IoT Work Together in Predictive Maintenance
IoT plays a major part by giving live data through connected devices like sensors or smart equipment. These gadgets track various metrics, such as temperature, vibrations, or pressure levels. AI then analyzes this information using advanced algorithms, which allow it to predict potential issues early.
AI, through machine learning, picks up on patterns that traditional systems often miss. For example, AI learns what typical operating conditions look like and alerts operators when something is off. This can prevent failures before they happen.
A real-world example of this is Rockwell Automation’s FactoryTalk Analytics GuardianAI. This system scans data from existing machinery, alerting operators before breakdowns occur. Since it doesn’t require installing extra sensors, it’s a cost-efficient way to make maintenance more proactive while also improving safety and minimizing interruptions.
The combination of AI with IoT forms a system that keeps learning and improving as more data is fed into it. Over time, these systems predict failures with more precision, leading to better-planned maintenance schedules and longer-lasting machines. For example, Nokia’s facility in Finland saw productivity jump by 30% after they rolled out AI-based maintenance and automated systems, showing how powerful this method can be.
Major Advantages of Predictive Maintenance
Less Downtime
Predictive maintenance reduces unexpected failures, making it easier to plan maintenance when it’s less disruptive. This helps production lines run smoothly and cuts down on expensive interruptions. Reports suggest these systems can reduce unscheduled downtime by up to 40%.
Cost Efficiency
Predicting equipment failures saves manufacturers from costly emergency repairs and lowers general maintenance expenses. This approach also reduces the need to stockpile spare parts, which can be costly to store and handle.
Longer Equipment Life
Regular predictive maintenance keeps machines running longer by addressing minor issues before they turn into major ones. This maximizes the return on investment as machinery lasts longer.
Improved Safety
Well-maintained equipment means fewer risks of accidents, which is crucial in industries like automotive or heavy machinery, where failures can lead to serious safety hazards.
Better Product Quality
Properly maintained machines perform better, leading to fewer defects in products. For instance, Samsung uses AI to uphold its high standards, which has resulted in higher customer satisfaction.
Real-World Applications and Examples
- General Electric (GE) is a great example. Their cloud platform, Predix, merges IoT and AI to oversee machinery in real time. In the healthcare space, GE uses this technology to predict when machines like MRI scanners may fail. As a result, they’ve lowered downtime and made critical medical equipment more reliable.
- Similarly, Nestlé has brought AI into its supply chain and maintenance processes. By predicting when machinery needs servicing, Nestlé has made its production smoother, cutting inefficiencies and improving output.
- Siemens also uses AI-driven predictive maintenance in its Digital Lighthouse plants. With real-time analytics from IoT devices, they can predict failures, which has helped cut downtime and boosted productivity.
- NVIDIA’s RAPIDS AI technology is another player in this field, providing a platform to speed up sensor data analysis. This allows manufacturers to react faster, reducing downtime by moving from hours of data processing to just minutes.
Challenges and Factors to Consider
Despite the obvious benefits, there are challenges when combining AI and IoT in manufacturing. One big concern is data security. The increased connectivity opens more avenues for cyberattacks, so manufacturers need strong protection measures. Moreover, the upfront costs of AI and IoT implementation can be high, though long-term savings typically make it worthwhile.
For newer machines without a lot of historical data, it can be hard to effectively train predictive models. However, advancements like data augmentation are helping fill in these gaps by simulating data when real-world examples are scarce.
Also, manufacturers need to make sure their workers are prepared for these advanced systems. As AI takes on more tasks, workers need new skills to manage and maintain them. Training programs, such as AI learning paths, help employees stay updated and better equipped to handle these systems.
Future Trends to Watch
Looking ahead, several trends are influencing AI’s future in manufacturing:
- Edge AI: Processing data close to its source (edge computing) is becoming more important. This reduces delays and makes decision-making faster, improving the efficiency of predictive maintenance.
- Digital Twins: Virtual models of real assets are becoming more common. These digital replicas allow companies to test scenarios without impacting actual operations.
- Improved Machine Learning: AI models are growing more advanced, making them better at predicting even complex failures, which improves reliability.
Conclusion
AI and IoT integration is changing predictive maintenance in manufacturing. These tools help businesses run more efficiently, cut costs, and ensure their machines stay reliable. As technology grows, AI’s role in manufacturing will become even more crucial, with predictive maintenance being key to modern industry.