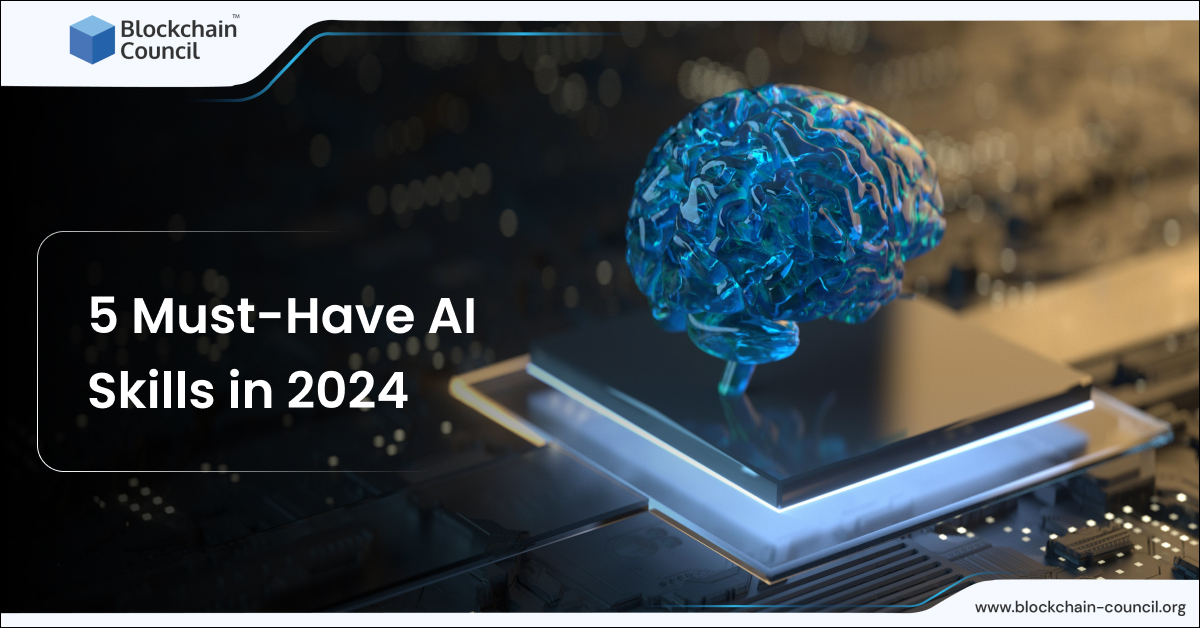
- Blockchain Council
- October 28, 2023
Summary:
- AI offers lucrative job opportunities with high salaries.
- Machine Learning (ML) is a fundamental concept in AI, teaching computers to learn from data and make predictions. Deep learning is a subset of ML.
- Recent trends in ML include multimodal learning, TinyML, AutoML, and MLOps.
- Deep learning is inspired by how the human brain works, making computers capable of understanding data like images, text, and sounds. It powers applications like voice-activated remotes and self-driving cars.
- Recent trends in deep learning include virtual assistants, image recognition, natural language processing, and transfer learning.
- Computer vision enables machines to interpret visual data and is crucial in various industries.
- Recent trends include generative AI, data-centric AI, merged reality, facial recognition, and enhanced 3D modeling.
- AI-powered cloud computing combines AI and cloud resources, making advanced AI applications accessible to various industries.
- Recent trends in AI-powered cloud computing include AI-powered cloud services, AI-optimized cloud infrastructure, multi-cloud AI, and edge AI.
Artificial Intelligence has emerged as one of the leading fields to offer lucrative job opportunities. From AI experts to NLP experts, every industry is in need of AI professionals. Companies like Netflix are offering over $300,000 to AI professionals. And the list goes on. 45% of the US population is already using AI. Its market is constantly growing. In fact, the global AI market is expected to reach $110.8 billion by 2030. As we stand on the verge of 2024, it is important to know the top 5 skills you will need to shine in an AI career in 2024. But what are the top AI skills that we should certainly keep an eye on in 2024?
Read till the end of this article to find the answers. In this article, we explore the 5 must-have AI skills in 2024. So, let’s dive in!
Machine Learning
Machine learning, or ML for short, is a fundamental concept in the world of artificial intelligence. It’s like the magic that powers many of the smart technologies we use daily, from voice assistants to recommendation systems. But what exactly is machine learning, and why is it so essential in today’s tech-driven world?
At its core, machine learning is about teaching computers to learn from data and experiences, just like how we humans learn from our mistakes and successes. The goal is to make these machines smart enough to spot patterns, make predictions, and even improve their performance without human intervention.
Imagine your favorite music streaming service, like Spotify. It seems to know your taste in music better than you do sometimes, right? That’s machine learning in action. It learns from your listening history, your likes, and dislikes, and then recommends songs you’ll love. This is just one small example of how ML can make our lives better and more convenient.
Now, how does it work? Unlike traditional programming where humans write out every step, machine learning takes a different approach. It starts with feeding data – lots of it – into a computer. This data can be anything from pictures and text to numbers. The more, the better.
Next, we choose a machine learning model, a kind of digital brain that will help make sense of this data. The machine learning model takes over and starts to learn. It searches for patterns, connections, and insights hidden within the data.
Think of it like teaching a dog new tricks. You show the dog a trick, and it keeps trying until it gets it right. In the same way, the machine learning model keeps learning and adapting, improving its performance as it processes more data.
Why is machine learning so important today? Well, it’s because we’re living in the era of big data. With the internet, smartphones, and countless sensors, we’re constantly generating a massive amount of data. Machine learning is the key to unlocking the potential of this data. It’s used in various fields, from finance and healthcare to entertainment and even self-driving cars.
You might have heard about deep learning, a subset of machine learning. It’s like taking machine learning to the next level. Deep learning is inspired by how our brains work and is exceptionally good at tasks like understanding language, recognizing faces, and more.
In summary, machine learning is the bridge between humans and computers. It allows us to teach machines to learn and make smart decisions based on data, opening up a world of possibilities. Whether you’re a beginner just dipping your toes into this field or a pro looking to stay ahead of the curve, understanding machine learning is a must.
So, next time you get a movie recommendation on Netflix or enjoy a chat with a helpful AI assistant, you’ll know that machine learning is the magic behind it all, making our digital world a little smarter every day.
Recent Trends and Developments
1. Multimodal Learning
Multimodal learning is an exciting frontier in machine learning. It enables machines to learn from various types of data, such as text, images, and audio simultaneously. This development is rapidly expanding, leading to innovative applications. For instance, in the medical field, multimodal learning is being used to create diagnostic tools that can analyze both medical images and patient data to detect diseases more accurately. Additionally, it’s revolutionizing the way AI assistants interact with humans, making it possible for them to understand and respond to language and gestures more naturally.
A notable example in this area is DeepMind’s Gato, a multimodal AI system with diverse capabilities, including visual reasoning and language comprehension. Gato’s versatility stems from being trained on a vast dataset that encompasses text, code, and images. It excels at quickly learning new tasks efficiently.
2. TinyML
TinyML is an emerging branch of machine learning designed to run on small, low-power devices, such as microcontrollers and sensors. The potential applications of TinyML are vast. It’s being harnessed to create wearable devices that monitor health and fitness, as well as smart home devices that adapt to our habits and preferences by automating tasks accordingly.
For instance, Google’s Coral offers a family of low-power AI accelerators tailored for TinyML applications. These Coral devices are versatile, capable of running machine learning models on various devices, including microcontrollers, Raspberry Pis, and mobile phones.
3. Automated Machine Learning (AutoML)
AutoML is simplifying the process of developing and deploying machine learning models. It provides a set of tools and techniques that automate many aspects of machine learning, from data preparation to model tuning. This accessibility empowers individuals without extensive machine learning expertise to build and deploy accurate models.
An excellent example of AutoML is Google Cloud AutoML, a cloud-based service offering pre-trained machine learning models for tasks like image classification, text classification, and object detection. It also enables users to train custom models without needing to write complex code.
4. Machine Learning Operationalization Management (MLOps)
MLOps is a set of best practices for organizations to efficiently deploy and manage machine learning models in a production environment. These practices, along with supporting tools and techniques, ensure that machine learning models are reliable, scalable, and secure.
For instance, Kubeflow is an open-source MLOps platform that simplifies deploying and managing machine learning models on Kubernetes. It streamlines the entire machine learning lifecycle, from development to deployment and monitoring, making it easier for organizations to harness the power of machine learning in real-world applications.
Also Read- Top 5 Artificial Intelligence Trends In 2024
Deep Learning
Deep learning is a part of artificial intelligence (AI). It’s a method inspired by how the human brain works. In deep learning, computers use complex models to understand data, like images, text, and sounds. These models can recognize patterns and make accurate predictions. This technology can be used to automate tasks that usually need human intelligence, like describing pictures or turning spoken words into text.
Artificial intelligence aims to make computers think and learn like humans. Deep learning is a big part of this. It’s behind everyday things like digital assistants, voice-activated remotes, and fraud detection. But it doesn’t stop there; deep learning is a key player in emerging technologies such as self-driving cars and virtual reality.
Deep learning models are like trained computer brains. Data scientists teach them how to do specific tasks using algorithms or predefined steps. These models are used by businesses to analyze data and make predictions in various applications. Deep learning is like having a smart assistant that learns from experience and makes decisions. It’s shaping the future of technology, making AI smarter and more capable.
Deep learning powers everyday AI things like voice-activated TV remotes, digital assistants like Siri and Alexa, and fraud detection. It’s also the magic behind emerging tech like self-driving cars and virtual reality. Businesses use deep learning models, which are files trained by data scientists, to crunch data and make predictions.
Recent Trends and Developments
Virtual Assistants
Virtual assistants like Siri, Alexa, and Google Assistant have become an integral part of our daily lives. These AI-powered assistants use deep learning to understand and respond to human language. Siri is featured in Apple devices, Alexa in Amazon devices, and Google Assistant in Google devices. They can perform tasks, answer questions, and make our lives more convenient.
Image Recognition
Deep learning has made significant advancements in image recognition. Companies like Tesla and Waymo use deep learning for self-driving cars, allowing them to recognize and react to their surroundings. Facial recognition software by companies such as Facebook and Google also relies on deep learning. Additionally, medical imaging benefits from deep learning, with companies like GE Healthcare and Philips Healthcare using it to improve diagnostics and patient care.
Natural Language Processing
Natural language processing (NLP) is a field where deep learning has had a profound impact. Machine translation services like Google Translate and Microsoft Translate use deep learning to provide accurate translations across languages. Chatbots developed by companies like Amazon and Google use deep learning to understand and respond to human conversations, making customer support more efficient. Social media analysis, performed by companies like Facebook and Twitter, utilizes deep learning to gain insights from the vast amount of data generated on these platforms.
Also Read- Why AI Is Important For The Metaverse
Machine Learning Vs Deep Learning
Aspect | Machine Learning | Deep Learning |
Neural Networks | Typically not used | Central component, uses neural networks |
Data Size | Can work with smaller datasets | Requires large datasets and computing power |
Feature Engineering | Often relies on manual features | Automatically learns features and representations |
Algorithm Complexity | Uses simpler algorithms | Employs highly complex algorithms like deep neural networks |
Interpretability | Generally more interpretable | Often less interpretable due to network depth |
Training Time | Faster training with smaller data | Longer training times, especially for deep networks |
Application Range | Suitable for a wide range of tasks | Excels in complex tasks, e.g., image and speech recognition |
Resource Requirements | Requires fewer computational resources | Demands substantial computing power |
Overfitting | Prone to overfitting with complex models | Better generalization with large datasets |
Transfer Learning | Less effective in transfer learning | Effective in transfer learning due to feature extraction |
Also Read- Fraud Detection Using Machine Learning
Natural Language Processing
NLP, or Natural Language Processing, is the technology that gives machines the power to understand and work with human language. It’s not just about recognizing words; it’s about comprehending context, semantics, and the intricate web of human communication. NLP equips machines to understand the way we speak, write, and even the underlying sentiment.
Let’s break it down. NLP enables machines to speak our language, both figuratively and literally. Think about voice-activated assistants, chatbots, and language translation tools. They’re all powered by NLP. It’s the magic that makes your smartphone understand your voice commands and respond intelligently. NLP is not just a shiny tool in the AI toolkit; it’s a game-changer. Its importance extends far beyond convenience, touching countless aspects of our daily lives.
Consider this: Conversational agents like Siri and Alexa rely on NLP to process your voice commands. They are like digital companions who understand your questions and provide meaningful answers. It’s the bridge between humans and machines, making interactions seamless and natural.
But NLP goes even deeper. It’s the force behind the scenes in search engines like Google, making sure you find exactly what you’re looking for. Social media platforms use it to detect and filter out hate speech, keeping online spaces safe. In the world of healthcare, NLP interprets electronic health records, making it easier for medical professionals to access crucial information.
The applications of NLP are boundless, and it’s only getting more sophisticated. However, challenges remain, like addressing biases and incoherence. Yet, these challenges present opportunities for those diving into the world of NLP.
Recent Trends and Developments
Multilingual Language Models
In the past, NLP models were often limited to one language, but that’s changing rapidly. Recent breakthroughs in multilingual NLP have given rise to models capable of handling multiple languages. Take Facebook’s M2M-100, for instance. This powerhouse can translate between an astonishing 100 languages, all without relying on English as an intermediary. It’s breaking down language barriers, enabling translations between less common language pairs, like Chinese and Swahili.
Large Language Models (LLMs)
These are the heavyweights of NLP. LLMs are trained on colossal datasets, equipping them with a diverse skill set. Google AI’s Bard LLM, for example, can do it all. From generating poems and code to writing scripts, musical pieces, emails, and letters, it’s a creative dynamo. It doesn’t stop there; it can tackle translation and answer questions comprehensively.
Transfer Learning
In the quest for efficiency, transfer learning has become a game-changer. NLP models can now be trained on one task and then applied to others. Think of it as a shortcut in AI development. For instance, a model initially designed to classify text as spam or non-spam can serve as the foundation for a model that classifies text by genre, such as news, sports, or entertainment.
Reinforcement Learning
It’s not just for gaming anymore. Reinforcement learning is being used to train NLP models by letting them interact with their environment and receive rewards for success. Imagine a model that generates text based on a query and is fine-tuned through interaction with a human user who provides feedback on its relevance.
Computer Vision
Imagine if machines could see and understand the world just as we do. Well, that’s what computer vision is all about. It’s the wizardry that enables computers to interpret the visual world, and it’s at the heart of Artificial Intelligence (AI).
In simple terms, computer vision equips machines with the power of sight. They can look at images, videos, and other visual inputs and make sense of them. Think of it as teaching a machine to see, observe, and comprehend.
But how does it work? Unlike humans who have a lifetime of learning, computers have to do it quickly. They don’t have eyes, retinas, and visual cortex. Instead, they rely on cameras, data, and algorithms.
Computer vision relies on two key technologies: deep learning and convolutional neural networks (CNNs). Deep learning is like the computer teaching itself to understand what it sees. The more data it processes, the smarter it gets. It learns to differentiate one image from another, much like we do.
CNNs are the backbone of this process. They break down images into tiny pixels, assign tags or labels to them, and make predictions. It’s a bit like recognizing shapes and edges first, just as we do when we see something from a distance, and then refining that understanding.
Computer vision is essential in numerous industries, from energy to manufacturing, and it’s expected to grow to new heights. So, why is computer vision crucial? Because it allows machines to do tasks that used to be purely human. They can inspect products, monitor processes, and spot tiny defects in a flash, surpassing human capabilities. It’s not just about seeing; it’s about understanding, and it’s changing the game in AI.
Recent Trends and Developments
Generative AI and Improved Training
Generative AI, a technology creating new content like images and text, is revolutionizing computer vision. Algorithms like Generative Adversarial Networks (GANs) produce synthetic training data, enhancing computer vision model learning. For instance, companies like Tesla utilize computer vision to develop self-driving cars that can perceive their surroundings and make navigation decisions in real-time.
Data-centric AI for Enhanced Performance
Data-centric AI, focusing on utilizing data to enhance AI systems, is pivotal for computer vision. Algorithms optimize accuracy, efficiency, and reduce necessary training data, critical for large-scale vision models. Google’s AI Pass is a prime example, using computer vision to enable passwordless login on Android devices, making the user experience more secure and convenient.
Merged Reality Applications
Merged Reality (MR) blends real and virtual worlds. MR headsets overlay digital information onto reality, creating innovative computer vision applications like real-time digital information display and interactive experiences. For instance, Apple’s Face ID uses advanced computer vision techniques to unlock iPhones and iPads seamlessly, showcasing the potential of MR technology.
Facial Recognition Technology
Facial recognition in computer vision identifies and tracks individuals through facial features. Widely applied in security, surveillance, and marketing, it raises privacy and ethical concerns while reshaping various industries. For example, Facebook harnesses computer vision to automatically identify and tag people in photos, transforming the way we interact with images on social media.
Enhanced 3D Modeling Capabilities
Computer vision algorithms are improving 3D model accuracy from images and videos, enabling new applications in robotics, manufacturing, and entertainment. Consider John Deere tractors, which use computer vision to guide themselves through fields and plant seeds with precision, enhancing agricultural efficiency.
Also Read- How To Use Google Bard?
AI-powered cloud computing
AI-powered cloud computing is the fusion of two powerful technologies, artificial intelligence (AI) and cloud computing. It’s like pairing a brilliant mind with an infinite toolbox. Cloud computing provides the necessary infrastructure – servers, storage, and more – delivered over the internet. AI, on the other hand, empowers machines to simulate human intelligence, enabling them to learn, adapt, and make decisions.
The magic unfolds when AI and cloud computing join forces. AI algorithms, with their ability to crunch vast amounts of data and draw intelligent insights, find a perfect home in the cloud. The cloud’s scalability, accessibility, and cost-efficiency create an ideal environment for AI’s data-hungry operations.
In simpler terms, AI in the cloud allows businesses to tap into the full potential of AI without the burden of setting up and maintaining complex infrastructure. This means even smaller enterprises can harness the capabilities of AI that were once reserved for tech giants.
AI-powered cloud computing isn’t just about convenience; it’s a catalyst for innovation. It makes sophisticated AI applications like machine learning and natural language processing accessible to a wide range of industries. From healthcare to finance, from retail to manufacturing, businesses can use AI in the cloud to enhance decision-making, automate routine tasks, and deliver smarter services to their customers.
Moreover, AI and cloud computing are a dynamic duo that evolves with the times. As AI algorithms get smarter, cloud computing resources become more abundant and cost-effective. This dynamic synergy ensures that you’re not just adopting a technology; you’re embracing a journey of perpetual improvement.
Here’s the key point: AI algorithms in the cloud are accessible to a wide range of industries and businesses. Whether you’re a healthcare provider, a financial institution, a retailer, or a manufacturer, AI in the cloud can enhance your decision-making, automate routine tasks, and improve customer services. It’s not just about convenience; it’s a catalyst for innovation, making advanced AI applications like machine learning and natural language processing within reach for all.
Recent Trends and Developments
AI-powered Cloud Services
Cloud providers are now offering intuitive AI-powered services. Take Google Cloud Platform, for instance. It provides AutoML, a service where businesses can train custom machine learning models seamlessly, without the complexity of coding. This means that even businesses without extensive technical expertise can harness the power of AI for their unique needs.
AI-Optimized Cloud Infrastructure
Cloud giants like Amazon Web Services have optimized their infrastructure for AI. They provide GPU-powered instances tailored for intensive tasks like training and running machine learning models. This optimization ensures that businesses can process vast amounts of data efficiently, enabling applications like real-time fraud detection for financial institutions.
Multi-Cloud AI
Today, many businesses are adopting a multi-cloud approach. Consider a scenario where a company trains its AI models using one cloud provider and deploys them in production with another. This flexibility ensures that businesses can cherry-pick the best features from different providers. For example, a retail company might leverage the robustness of AWS for training its models and use Microsoft Azure for seamless production deployment, enhancing both efficiency and scalability.
Edge AI
Edge AI, an emerging trend, brings AI capabilities closer to devices and sensors. Imagine a self-driving car making split-second decisions based on real-time data analysis at the edge. Cloud providers are facilitating this through edge AI services. These services empower businesses to deploy AI applications at the edge, ensuring rapid response times. This is particularly crucial in applications like smart factories, where immediate decision-making can optimize production processes and minimize downtime.
How to Learn Artificial Intelligence? Get Certified Today!
Certification Name | Benefits | Duration | Certification Validity | Exam | Access Mode |
Certified Artificial Intelligence (AI) Expert™ | Beginner-friendly training in AI and data science | 12 Hours | Lifetime | Online | Self-paced |
Covers core AI concepts and technologies | |||||
Up-to-date knowledge in the AI field | |||||
Certified Prompt Engineer™ | Learn prompt engineering and its impact | 6 Hours | Lifetime | Online | Self-paced |
Hands-on experience with OpenAI API | |||||
Cutting-edge techniques in prompt engineering | |||||
Certified ChatGPT Expert | Unlock career opportunities in ChatGPT and AI fields | 7 Hours | Lifetime | Online | Self-paced |
Master the revolutionary ChatGPT technology | |||||
Certified Artificial Intelligence (AI) Developer™ | Uplift your career as an AI developer | 14 Hours | Lifetime | Online | Self-paced |
Comprehensive training on AI concepts and core questions | |||||
Certified Chatbot Expert™ | Gain a comprehensive understanding of chatbot design | 6 Hours | Lifetime | Online | Self-paced |
Develop skills in natural language processing | |||||
Master the Art of AI (Learning Path) | Combine multiple AI certifications for a comprehensive | 38 Hours | Lifetime | Online | Self-paced |
4-step AI education path | |||||
Deep understanding of key AI concepts and techniques |
Conclusion
In conclusion, the world of AI is a high-reward, high-demand field. Netflix’s generous offer is just the tip of the iceberg, as industries worldwide seek prompt engineers to optimize their operations. To excel in this role, mastering automation, scripting, error handling, communication, and problem-solving are paramount. These skills will not only unlock a lucrative career but also ensure you remain at the cutting edge of prompt engineering. So, seize the opportunity, hone your abilities, and become the AI professional the world is waiting for.
FAQs
1: What is machine learning (ML) and why is it important in AI?
- ML is about teaching computers to learn from data and experiences.
- It’s important in AI because it enables machines to make predictions, recognize patterns, and improve their performance without human intervention.
- ML is the foundation of various AI applications like recommendation systems and digital assistants.
2: How is deep learning different from machine learning (ML)?
- Deep learning is a subset of ML, inspired by how the human brain works.
- It’s exceptionally good at tasks like understanding language, recognizing faces, and more.
- Deep learning uses complex models and neural networks, making it suitable for complex tasks like image and speech recognition.
3: What is natural language processing (NLP), and why is it important in AI?
- NLP equips machines to understand and work with human language.
- It goes beyond recognizing words, comprehending context, semantics, and human communication intricacies.
- NLP is crucial in applications like voice-activated assistants, search engines, social media analysis, and healthcare.
4: What is computer vision, and how does it work in AI?
- Computer vision enables machines to interpret visual data like images and videos.
- It works by using deep learning and convolutional neural networks to analyze and understand visual inputs.
- It’s essential in various industries, from manufacturing to energy, surpassing human capabilities in tasks like product inspection and process monitoring.
5: How does AI-powered cloud computing benefit businesses?
- AI-powered cloud computing combines AI’s data-processing capabilities with cloud infrastructure’s scalability.
- It makes advanced AI applications like machine learning and natural language processing accessible to a wide range of industries.
- It’s a catalyst for innovation, enhancing decision-making, automating tasks, and improving customer services without the need for complex infrastructure setup.