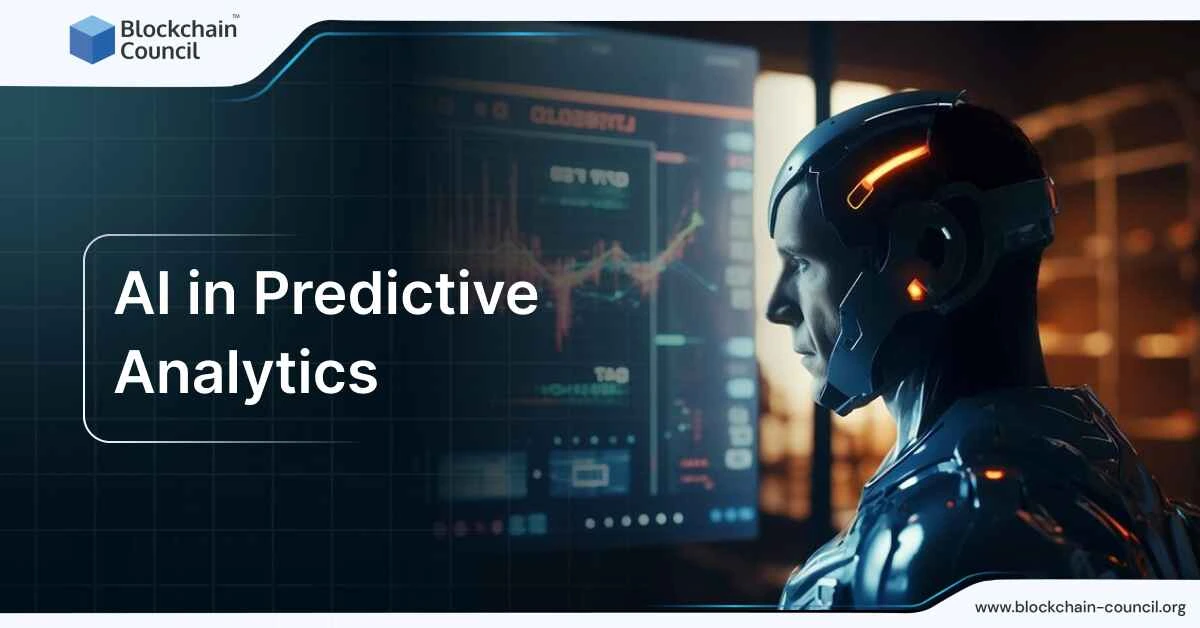
- Blockchain Council
- December 02, 2024
Artificial Intelligence (AI) has brought big changes to predictive analytics, helping organizations to foresee future patterns and make smarter choices. By studying past data, AI models can predict outcomes, spot patterns, and streamline processes across various fields.
Grasping Predictive Analytics
Predictive analytics involves applying statistical methods and machine learning to explore historical data and forecast upcoming events. By finding patterns and trends, businesses can make informed choices, reduce risks, and take advantage of new opportunities. AI strengthens predictive analytics by handling vast amounts of data quickly and accurately, revealing insights that humans might miss.
To gain deeper insights into predictive analytics, the Certified Artificial Intelligence (AI) Expert™ certification is a great option.
AI in Healthcare
In healthcare, AI-powered predictive analytics has greatly boosted both patient care and operational efficiency. For example, Eastman, a global leader in specialty materials, has added generative AI to its data framework to improve functionality. By concentrating on its core strengths, Eastman developed a digital layer offering smart services. One key feature is predicting when heat transfer fluids, used by clients, might degrade, aiding preventive maintenance and minimizing downtime.
Additionally, AI helps forecast disease outbreaks by analyzing data from social media, health records, and other sources. This assists healthcare providers in managing resources wisely and acting on preventive measures quickly.
Financial Sector
In finance, AI-driven predictive analytics is used to assess credit risks, spot fraud, and refine investment strategies. For instance, a study launched MarketSenseAI, an advanced model using GPT-4 to choose stocks based on market trends, news, economic factors, and other data points. MarketSenseAI mimics expert decision-making and showed impressive results in tests, offering higher returns on the S&P 100 stocks over 15 months, reaching up to a 72% cumulative return while maintaining a balanced risk level.
AI models also study transaction behavior to detect unusual activity, signaling possible fraud and helping banks act quickly to safeguard funds.
Retail
Retailers use AI-based predictive analytics for inventory management, demand forecasting, and creating personalized customer experiences. For instance, the fashion industry faces significant challenges with excess inventory, costing billions annually in unsold stock. AI-powered tools are becoming a solution, with startups offering technology to improve stock levels and predict demand. Autone, a London-based startup, has raised $17 million to develop AI tools for actionable inventory insights. Other firms like Singuli and Prediko offer similar solutions aimed at reducing waste and boosting efficiency.
AI also examines customer habits to deliver tailored recommendations, enhancing customer satisfaction and loyalty.
To fully understand AI’s predictive power, the Master Artificial Intelligence (AI) Learning Path offers comprehensive guidance.
Manufacturing
In manufacturing, AI-based predictive analytics supports maintenance prediction and quality management. For example, Qantas has been using AI since 2018 to fine-tune parts of its operations like flight routes, fuel supply, and managing external factors, leading to fuel savings of around $92 million. AI applications in aviation also extend to route planning, fuel efficiency, and maintenance prediction.
Moreover, AI models keep an eye on production processes to catch irregularities, ensuring steady product quality and minimizing waste.
Government and Public Safety
Government organizations use AI-powered predictive analytics to advance public safety and resource management. For example, U.S. intelligence agencies are starting to use generative AI to handle the growing volume of data and stay competitive. Even with its advantages, officials are mindful of AI’s limitations, like the risks of errors and security concerns. Rhombus Power’s AI model, for instance, predicted Russia’s invasion of Ukraine with 80% accuracy. Generative AI tools like Osiris, a tool developed by the CIA, summarize unclassified information but are used with caution, keeping human analysts at the core of intelligence work.
AI also analyzes crime statistics to foresee potential hotspots, enabling law enforcement to assign resources more effectively and reduce crime rates.
Obstacles and Cautions
Despite the benefits, AI-driven predictive analytics brings challenges. Concerns about data quality and privacy are central, as flawed or biased data could lead to incorrect predictions. Additionally, the complex nature of AI models may make it hard to explain how decisions are made, leading to a lack of transparency. Businesses need to manage these issues by maintaining data accuracy, setting ethical standards, and promoting clarity in AI use.
For anyone interested in refining AI responses in predictive models, becoming a Certified Prompt Engineer™ could be a solid path.
Wrapping Up
AI has changed predictive analytics, giving organizations valuable tools to anticipate trends, streamline processes, and make thoughtful choices. From healthcare to finance, retail to manufacturing, and public safety, AI-powered predictive analytics is making a positive impact across many fields. As technology moves forward, it’s essential for organizations to adopt AI thoughtfully, addressing challenges and making the most of its potential to support progress and achievements across various sectors. A Certified Artificial Intelligence (AI) Developer™ credential can provide the technical skills needed to build predictive AI models.