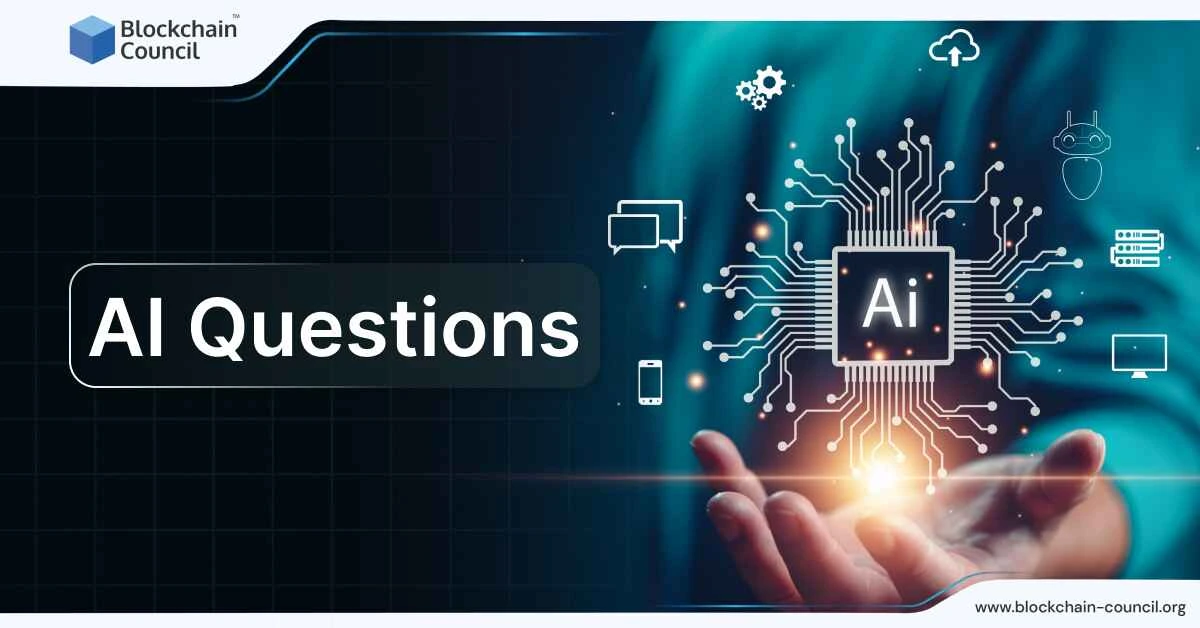
- Blockchain Council
- October 03, 2024
Artificial Intelligence (AI) can seem confusing, especially if you’re new to the subject. Many beginners have specific questions about understanding AI basics.
What Is AI?
Artificial Intelligence (AI) refers to technology designed to perform tasks that typically require human intelligence. These tasks could be decision-making, solving problems, understanding spoken words, or recognizing visuals. For example, when your phone suggests the next word while you’re typing or when a streaming service offers movie recommendations, that’s AI functioning behind the scenes.
How Does AI Operate?
AI works by mimicking how people think and solve problems, usually through processing huge sets of data. One major method AI uses is called machine learning (ML), where the system improves through practice. For example, you can train a computer to recognize images of cats. You show it hundreds of photos of cats, and over time, it “learns” what characteristics make up a cat, like ear shape or fur color. This process of teaching a computer, known as model training, is a key part of machine learning.
Types of AI
AI is generally divided into two categories: narrow AI and general AI.
- Narrow AI: This type is highly skilled at one specific task but can’t perform anything beyond that. For example, a chatbot programmed to help with electricity bills can’t also cook a meal or clean a house.
- General AI: This form would be able to complete any intellectual task a human can do. However, this kind of system does not yet exist and remains a concept at this point in time.
What Is Deep Learning?
Deep learning is a part of machine learning that works using layers, similar to how the brain’s neural networks function. These layers, also called neural networks, allow AI to manage large amounts of data and find patterns. For instance, deep learning helps with image recognition. When you upload a picture on social media, AI categorizes it by tagging people or identifying objects. As AI handles more data, it becomes better at sorting and predicting information.
Examples of AI in Use
AI is widely used across different industries for practical applications. Here are a few common examples:
- Voice Assistants: Devices such as Alexa, Siri, and Google Assistant utilize AI to interpret and respond to voice commands.
- Healthcare: AI assists doctors by analyzing medical images or predicting results based on patient data.
- Self-driving Vehicles: AI allows these cars to read traffic signals, assess road conditions, and identify obstacles, helping them move without needing human control.
What Is AI Bias?
AI bias happens when the data used for training a system is not balanced, causing unfair or inaccurate outcomes. For example, if an AI hiring system was trained with biased data, it might unfairly favor candidates from certain backgrounds. Solving AI bias means carefully collecting and testing data to ensure fair results. Using a diverse set of data can help reduce bias and create fairer systems.
What Does Explainable AI Mean?
Explainable AI (XAI) aims to make AI’s decisions more understandable to humans. As AI becomes more complex, its decision-making process can seem unclear, like a “black box,” where users don’t fully understand why a certain decision was made. Explainable AI tries to provide insights into how decisions are reached, which helps in building trust. This is especially important in sectors like healthcare and finance, where clear and transparent results are essential.
What Are Overfitting and Underfitting?
Overfitting takes place when a model becomes too fixated on the training data, including irrelevant patterns, making it hard to work with new information. It’s similar to focusing on memorization without understanding the overall concepts. In contrast, underfitting occurs when the model doesn’t learn enough from the data, leading to underwhelming results.
To prevent overfitting, methods like regularization and cross-validation are used. In cases of underfitting, developers may increase the complexity of the model or add more training data.
What are the Challenges of AI?
Despite its potential, AI has certain hurdles:
- Data Privacy: AI depends on vast amounts of data, which brings up worries about safeguarding personal or sensitive information. It’s important for AI systems to comply with rules like GDPR to protect user data.
- Explainability: As discussed earlier, understanding how AI arrives at its decisions can be difficult, particularly with complex deep learning models. This is a crucial issue in high-stakes sectors such as healthcare, where people need to trust AI’s suggestions.
- Bias: When AI is trained on biased data, it can lead to results that are unfair or incorrect. For example, facial recognition systems have been shown to perform worse with people who have darker skin, due to biased training data.
- Costs and Resources: AI needs a lot of computing power, which can be costly to set up and maintain.
Ethics in AI
Ethics in AI is a growing concern. It involves making sure AI systems are fair, transparent, and do not violate anyone’s rights. For instance, when AI is used in law enforcement or surveillance, it raises questions about privacy and potential bias. To address these issues, many companies are setting up internal guidelines and committees to ensure their AI systems are ethically developed.
Conclusion
Artificial Intelligence is influencing many areas of life, from the way businesses operate to how individuals interact with technology. Although it comes with certain challenges like bias, transparency issues, and ethical concerns, AI continues to grow and bring new possibilities. By learning how AI operates and tackling its issues, we can create more reliable systems that benefit everyone.