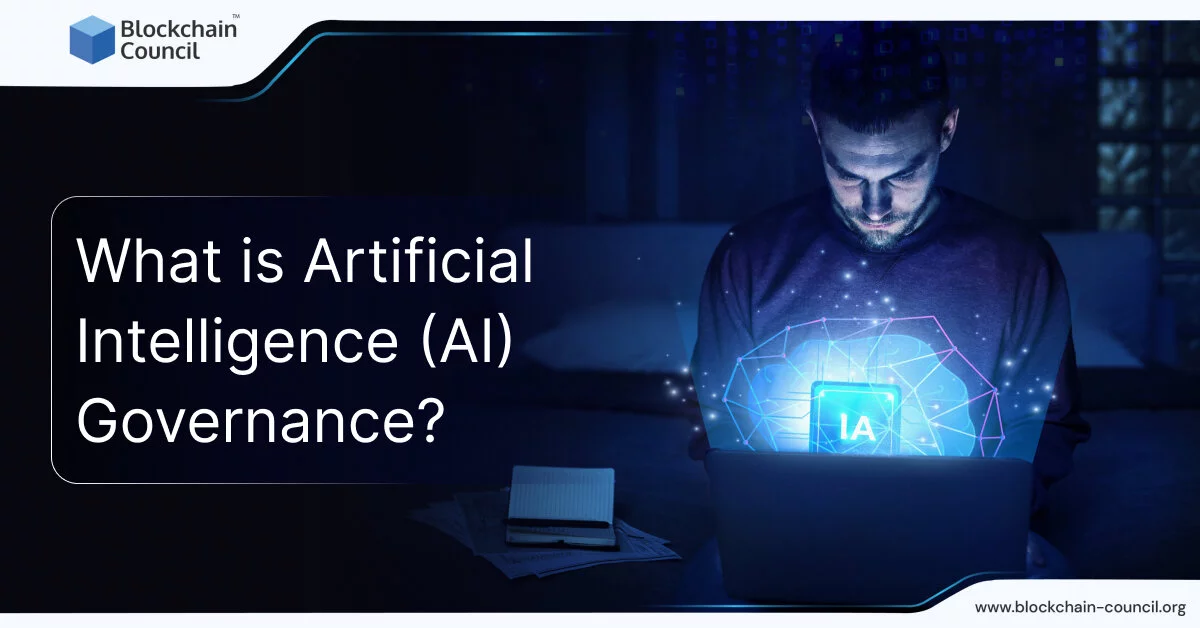
- Blockchain Council
- September 13, 2024
Summary
- AI Governance involves strategies to guide the ethical development, deployment, and management of AI technologies.
- It aims to ensure AI systems operate safely, transparently, and accountability while safeguarding human rights.
- AI governance is essential as AI becomes integrated into various aspects of society.
- Early AI development lacked comprehensive oversight, leading to the need for ethical guidelines.
- International organizations like OECD and EU played a role in shaping early AI governance frameworks.
- National governments, such as the US, developed their AI strategies and guidelines.
- AI governance evolved from principles to concrete regulatory frameworks.
- China, the US, and the EU have different approaches to AI governance.
- Challenges include balancing innovation with regulation, addressing bias in AI systems, and ensuring global cooperation.
- The future of AI governance includes trends like generative AI in media, AI-generated disinformation, multitasking robots, and integration with data governance.
Artificial Intelligence (AI) Governance refers to the framework and strategies employed to guide the ethical development, deployment, and management of AI technologies. It encompasses a range of policies, principles, and practices designed to ensure that AI systems operate in a safe, transparent, accountable, and equitable manner. AI governance aims to address the complex ethical, legal, and social implications that arise from the use of AI, balancing innovation and regulation while safeguarding public interest and fundamental human rights.
In today’s rapidly evolving technological landscape, AI governance has become increasingly crucial. AI systems are becoming more integrated into various aspects of society, from healthcare and education to finance and transportation. The pervasive nature of AI presents unique challenges such as potential biases, privacy concerns, ethical dilemmas, and the need for transparency and accountability. Effective AI governance is vital to harness the benefits of AI technologies while minimizing their risks and adverse impacts. It ensures that AI advancements contribute positively to societal development and are aligned with human values and ethical standards.
In this article, we provide a comprehensive overview of AI governance. So, let’s dive in!
Historical Context of AI Governance
Early Stages of AI Development and the Need for Governance
The inception of artificial intelligence (AI) ignited a technological revolution, but it soon became evident that this powerful tool needed governance. The early stages of AI development were marked by rapid advancements without comprehensive oversight, raising concerns about ethical use, safety, privacy, and the impact on society.
AI governance initially focused on establishing ethical guidelines and principles. International organizations like the OECD, EU, and UNESCO played a pivotal role in shaping these early frameworks. The OECD’s “Principles on Artificial Intelligence” (2019) and the EU’s “Ethics Guidelines for Trustworthy AI” (2019) are notable examples. These frameworks aimed to ensure safe, transparent, and ethical AI development, emphasizing accountability and human rights.
National governments also contributed by developing their own AI strategies and guidelines. The US, for instance, released the “AI Risk Management Framework” by the National Institute of Standards and Technology (NIST) in 2023 and the “Blueprint for an AI Bill of Rights” in 2022. Such documents provided guidance for regulators and policymakers, reflecting a growing international commitment to responsible AI development.
Evolution of AI Governance Over Time
As AI technology evolved, so did its governance. The field transitioned from principle-based guidelines to more concrete regulatory frameworks. The EU AI Act, proposed in 2023, exemplifies this shift, aiming to establish a human-centric framework for AI usage. This act classifies certain AI practices as unacceptable or high-risk, addressing issues like predictive policing and untargeted facial recognition. Similarly, the US proposed the “Algorithmic Accountability Act” and the “AI Disclosure Act” in 2023, reflecting a growing need for legislation in this domain.
China, another major player in AI, launched several principles and regulations, such as the “Global AI Governance Initiative” and measures for managing generative AI services. These developments underscore the global recognition of AI’s impact and the need for comprehensive governance.
However, operationalizing AI governance (AIG) at the organizational level remains a challenge. While various tools and processes have been proposed, such as ethics-based auditing and impact assessments, there is still a lack of clarity on stakeholder roles and effective implementation strategies. Research in this area is still in the early stages, indicating the need for more in-depth studies to develop functional AIG frameworks that can translate policy into practice effectively.
Key Principles of AI Governance
1. Transparency and Accountability
Transparency in AI refers to the clarity and openness in the development and deployment of AI systems. It involves making the processes, decisions, and impacts of AI applications clear and understandable to stakeholders. Accountability ensures that those who develop and deploy AI systems are responsible for their functioning and the ethical implications of their use. The AI Bill of Rights emphasizes the importance of providing clear notice and explanations of AI outcomes, ensuring that automated decision-making processes are transparent and accountable.
2. Fairness and Non-discrimination
AI systems must be designed to be fair, using representative data and proactive measures to ensure equity. This principle combats algorithmic discrimination, addressing biases that may arise due to training data or algorithms. The AI Bill of Rights mandates protections against biased or discriminatory AI algorithms, promoting fairness in AI technology and prohibiting bias based on gender, race, ethnicity, or other protected characteristics.
3. Safety and Security
AI systems should be safe and effective, with rigorous pre-deployment testing, independent evaluation, and ongoing monitoring to protect users from harm. This principle is highlighted in the AI Bill of Rights, which stipulates that individuals should be safeguarded from unsafe or inefficient automated systems. It also focuses on ensuring the safety and security of AI systems, reducing risks of accidents, failures, and inaccuracies.
4. Privacy and Data Protection
Respecting data privacy is crucial in AI governance. The AI Bill of Rights outlines privacy protections that prioritize design choices to safeguard against abusive data practices. It emphasizes the need for user consent for data collection and use, enhanced protections for sensitive data, and prohibitions on unchecked surveillance. This principle aligns with international data protection standards, such as the EU’s General Data Protection Regulation (GDPR).
5. Ethical Considerations and Human Values
Ethical AI involves aligning AI systems with human values and ethics. This includes prioritizing human alternatives and fallbacks in AI systems, ensuring accessibility, protection from harm, and timely human consideration and remedy. The AI Bill of Rights ensures that human alternatives are available and considered, allowing individuals to opt out of automated systems when necessary. This principle aims to reduce potential harms and unexpected consequences of AI, maintaining a human-centric approach in AI development and use.
Global Perspectives on AI Governance
Different Approaches by Leading Countries: USA, China, EU
- United States: In the U.S., AI governance is decentralized, with various federal agencies adapting to AI without new legal authorities. The approach is highly distributed, focusing on non-regulatory infrastructure, such as AI risk management frameworks and evaluations of facial recognition software. Comprehensive AI legislation is slow, with a fragmented approach spread across voluntary recommendations and non-binding regulations. Despite this, targeted federal laws are emerging on urgent issues like algorithmic bias and data privacy.
- China: China’s governance of AI blends state control with a drive to promote AI innovation. The approach is more vertical, using discrete laws to address specific AI issues, such as AI-driven recommendation algorithms and deep synthesis tools. China’s regulations also focus on data security, operational risk, and intellectual property concerns, with stringent measures for content moderation and algorithmic transparency. The country’s strategy aims to become a global leader in AI by 2030, balancing innovation with state oversight.
- European Union: The EU’s approach is comprehensive, with legislation tailored to specific digital environments. The EU AI Act, passed in early 2023, takes a risk-based approach, establishing categories for AI systems based on risk levels. It introduces stringent requirements for high-risk AI systems, transparency requirements for limited-risk systems, and minimal regulations for low-risk AI. The EU AI Act also establishes the European Artificial Intelligence Board for harmonized application across the EU.
- The U.S. and EU share conceptual alignment on a risk-based approach and trustworthy AI principles. Collaborative efforts, especially through the EU-U.S. Trade and Technology Council, have been successful in developing common understanding and metrics for trustworthy AI. These collaborations are foundational to the democratic governance of AI and include shared documentation, research, and knowledge sharing on standards development and AI assurance ecosystems.
Challenges in AI Governance
Balancing Innovation and Regulation
AI governance faces the challenge of balancing the need for innovation with the requirement for regulation. This balance is crucial to maximize the benefits of AI while minimizing risks. However, the challenge lies in creating regulations that do not stifle innovation. For instance, in the United States, the approach to AI risk management is highly distributed across federal agencies, and there is a focus on non-regulatory infrastructure such as AI risk management frameworks. This fragmented approach can sometimes lead to inconsistencies and may not always effectively address the rapid developments in AI.
In contrast, the European Union’s AI Act aims to provide a comprehensive legislative scheme governing AI, classified into risk categories and imposing stringent requirements on high-risk AI systems. This act illustrates a more centralized approach to AI governance. However, overly strict regulations may hinder innovation and the adoption of AI technologies.
Addressing Bias and Fairness in AI Systems
Bias and unfairness in AI systems is a significant challenge. AI can inadvertently perpetuate existing biases or create new forms of discrimination. For example, the use of proxies in data sets can lead to skewed outcomes, and old or incomplete data can contain hidden biases. Inaccurate or imprecise labeling of data can also introduce bias, affecting the fairness of AI applications. Addressing these issues requires a focus on data quality, performance modeling, and stress testing to identify and mitigate biases in AI systems.
AI systems’ fairness remains an art rather than a precise science. Data scientists and developers must consider the potential for misunderstandings and misapplications of proxies and labels. Furthermore, the balance between demographic parity and individual differences must be carefully managed to ensure fairness both at the group and individual levels.
Ensuring Global Cooperation and Standards
International cooperation on AI is vital to harmonize standards and regulatory approaches, which benefits all stakeholders involved in AI development and deployment. Since 2017, when Canada adopted a national AI strategy, at least 60 countries have formulated policies on AI. Global corporate investment in AI has reached significant levels, necessitating international standards and cooperation. Such collaboration can lead to more effective and uniform regulation, reducing barriers to innovation and diffusion.
However, aligning various international AI governance efforts remains challenging. Different countries have diverse priorities and approaches to AI governance. The U.S. executive order on AI and the AI Bill of Rights, for example, emphasize the need for international cooperation to make domestic AI governance efforts more effective. These include facilitating the exchange of AI governance experiences and broadening global access to computing power and data essential for AI development.
Case Studies and Real-world Examples
Case Study 1: Microsoft’s AETHER Committee
Microsoft established the AETHER (AI, Ethics, and Effects in Engineering and Research) Committee to address normative questions related to AI. This initiative highlights the importance of executive-level support in shaping an organization’s commitment to responsible AI development. It underscores the need for engagement with employees, experts, and integration with the company’s legal team. The AETHER Committee represents a model for large multinational companies to adopt new practices and oversight committees to ensure their technologies will be beneficial and ethically responsible.
Case Study 2: OpenAI’s Staged Release
OpenAI’s “staged release” of a powerful language processing model illustrates a shift in traditional software publishing norms. This approach was adopted to promote research and dialogue about possible harms associated with AI technologies. It represents a proactive measure in AI governance, focusing on accountability and responsible dissemination of AI advancements. OpenAI’s case is a significant example of how companies can use documentation efforts, research paper discussions, and communication strategies to manage potentially harmful uses and impacts of AI models.
Case Study 3: Organisation for Economic Co-operation and Development’s AI Policy Observatory
The Organisation for Economic Co-operation and Development (OECD) launched the AI Policy Observatory as part of an international effort to establish shared guidelines around AI. This initiative demonstrates the significance of international coordination and cooperation on AI. The OECD AI Principles are leveraged to support partnerships, multilateral agreements, and the global deployment of AI systems, emphasizing the need for a common understanding and desired outcomes for AI’s future.
Case Study 4: AuroraAI – Ethical AI in Public Sector
The case of AuroraAI in Finland exemplifies the ethical use of AI in public services. This case study emphasizes the importance of public engagement in AI deployment, focusing on transparency, agency, accountability, and fairness. It also highlights the challenges of public understanding and engagement with AI ethics, including the pace of technological change, complexity, and the need to debunk myths and misconceptions about AI.
Case Study 5: EOS at Federated Hermes – AI Ethics and Data Governance
EOS at Federated Hermes launched a project in 2019 to help companies understand the risks associated with AI and data governance. This case study underscores the importance of transparency in how companies use Big Data and machine learning. It advocates for companies to commit to overseeing the respect of all human rights and to publish AI principles and white papers that address legal, compliance, and technical concerns. This example demonstrates how private sector companies can develop a risk-aware culture and promote responsible AI and data governance.
The Future of AI Governance
Emerging Trends and Developments
The future of AI governance is shaping up to be a complex landscape with several key trends and developments:
- Generative AI in Media and Entertainment: The utilization of generative AI in the media and entertainment industry is rapidly advancing. Companies like Runway are developing generative video models that produce high-quality short videos, with applications ranging from filmmaking to marketing. Major movie studios are exploring the use of generative AI for lip-syncing actors’ performances in foreign-language overdubs and for special effects. This advancement raises serious questions about the role of actors and the ethical implications of AI in content creation.
- AI-Generated Disinformation: AI-generated disinformation, particularly in the political arena, is poised to become a significant challenge. The ease of creating deepfakes and realistic AI-generated content could greatly impact the political climate and the authenticity of online information. This trend underscores the need for robust mechanisms to track and mitigate AI-generated fake news and the importance of ethical considerations in AI development.
- Multitasking Robots: Inspired by techniques behind generative AI’s boom, the development of general-purpose robots capable of performing a wide range of tasks is on the rise. These advancements in robotics point towards a future where AI and robots become more integrated into various aspects of daily life and work, requiring comprehensive governance strategies.
- Data and AI Governance Integration: The definition of data is expanding, influenced by the integration of generative AI. This expansion necessitates a reevaluation of data governance standards, focusing on feature stores, model management, and data sharing. It highlights the need for governance frameworks that can adapt to the dynamic nature of data and AI processes.
- Intelligent Data Platforms: Predictions for 2024 suggest the emergence of intelligent data platforms that converge governance for AI and data. This trend indicates a move towards platforms that can manage the complexities of modern data ecosystems, integrating AI governance and data governance more closely.
Conclusion
AI governance is a critical aspect of contemporary technological advancement. It plays a pivotal role in ensuring that AI developments are ethical, responsible, and beneficial to society as a whole. As AI continues to evolve and become more ingrained in our daily lives, the importance of robust AI governance frameworks cannot be overstated. This article underscores the need for ongoing dialogue, international collaboration, and proactive policy-making to address the dynamic challenges posed by AI. Ultimately, the future of AI governance will significantly influence how AI shapes our world, making it essential for stakeholders across sectors to engage actively in its discourse and implementation.
Frequently Asked Questions
What is AI governance?
- AI governance refers to the framework and strategies used to guide the ethical development, deployment, and management of AI technologies.
- It encompasses policies, principles, and practices aimed at ensuring that AI systems operate safely, transparently, accountably, and equitably.
- AI governance addresses complex ethical, legal, and social implications arising from AI use while balancing innovation and regulation.
- It is vital for minimizing risks and adverse impacts of AI while harnessing its benefits for societal development.
What is the role of AI in good governance?
- AI can enhance transparency and efficiency in government processes by automating routine tasks, data analysis, and decision-making.
- AI-driven data analysis can help policymakers make informed decisions and predict trends, contributing to better governance.
- It can improve public services by providing personalized recommendations and streamlining interactions between citizens and government agencies.
- AI can also help in identifying and addressing issues like fraud, corruption, and inefficiencies, promoting good governance practices.
How can AI improve healthcare governance?
- AI can assist in healthcare governance by analyzing vast amounts of medical data, aiding in disease diagnosis, treatment planning, and monitoring patient outcomes.
- It helps in resource allocation, optimizing hospital operations, and reducing healthcare costs while maintaining quality care.
- AI-powered predictive analytics can identify potential outbreaks, enabling proactive measures for public health governance.
- Healthcare governance can benefit from AI-driven telemedicine, making healthcare services more accessible and efficient.
What are the challenges in implementing AI governance at the organizational level?
- Defining clear roles and responsibilities for stakeholders in implementing AI governance within an organization remains a challenge.
- Organizations struggle with developing effective AI governance frameworks that can translate policies into practical implementation.
- Ethical considerations and ensuring that AI aligns with human values can be complex to address in practice.
- Balancing innovation and regulatory compliance within an organization can be challenging, as overly strict regulations may hinder AI development and adoption.