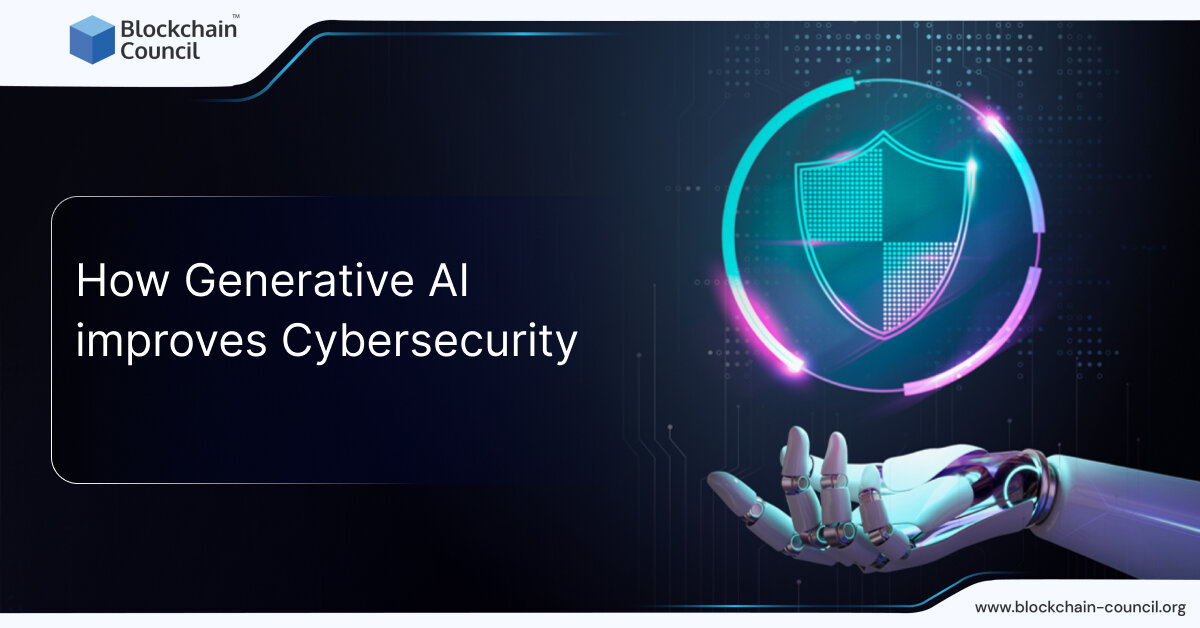
- Blockchain Council
- September 13, 2024
Summary
- Generative AI is a crucial advancement in cybersecurity, countering evolving cyber threats with its ability to analyze patterns and adapt.
- Its collaborative neural networks generate authentic data, mimicking human behavior and thinking, making it highly effective in cybersecurity.
- Generative AI enhances incident response, aiding in swift and accurate decision-making during security breaches.
- It continuously refines its understanding of threats, improving its predictive capabilities.
- Generative AI applications span various industries, such as healthcare, finance, transportation, e-commerce, and government.
- In cybersecurity, Generative AI excels in malware detection, phishing email detection, anomaly detection, and more.
- It provides enhanced threat intelligence, automates security tasks, and is cost-efficient and scalable.
- Ethical considerations, including data privacy and consent, are crucial in responsible Generative AI integration.
- Overcoming data scarcity and adversarial attacks are challenges addressed by Generative AI.
- Generative AI’s explainable approach builds trust, promising a safer digital future for cybersecurity.
Introduction
In our digitized world, cybersecurity is paramount. Threats loom, from data breaches to malware attacks. Every entity, be it a government, a business, or an individual, is vulnerable. Cyberattacks have become sophisticated, evolving constantly, making traditional defense mechanisms inadequate. Cybersecurity encompasses technologies, processes, and practices to protect digital systems and data. It’s a proactive strategy against potential breaches. AI has given cyber attackers a powerful tool, enabling automation and precision in their malicious activities.
This has elevated the need for a more advanced defense strategy. It is an advanced branch of AI. It’s designed to simulate and generate human-like data. In the realm of cybersecurity, this technology is a game-changer. This innovative approach significantly bolsters a system’s defenses. Generative AI analyzes patterns in data, learning from them to create realistic scenarios. It predicts potential cyber threats by understanding how attackers operate.
Moreover, Generative AI provides invaluable support in incident response, aiding in rapid and accurate decision-making during a security breach. By automating and enhancing incident analysis, it enables a swifter and more effective response, minimizing damage and recovery time. This efficiency can be the difference between a minor incident and a catastrophic security breach. One of the key strengths of Generative AI lies in its ability to adapt and self-improve. It continuously refines its understanding of threats and vulnerabilities, enhancing its predictive capabilities. In this article, we will discuss how Generative AI can improve cyber security.
Understanding Generative AI
Generative AI, or Generative Adversarial Networks (GANs), represents an innovative leap in artificial intelligence. It’s a technology where two neural networks collaborate: one generates data, and the other evaluates it. This collaboration fosters the creation of remarkably authentic data, mimicking human-like patterns. At its core, Generative AI has a primary goal – to create something new from scratch, be it images, texts, or even strategies. It’s a fusion of creativity and technology, simulating the way humans think and create. This AI’s fundamental concept is based on an adversarial process, constantly improving itself to produce results that are incredibly close to reality.
Applications in various industries
Healthcare Industry:
Generative AI augments cybersecurity within the healthcare sector by fortifying patient data privacy and ensuring compliance with stringent regulations like HIPAA. It streamlines data management, aiding in secure storage and analysis of medical records. Additionally, it enhances anomaly detection, flagging any suspicious activities in real-time, thereby protecting the integrity of patient information.
Finance and Banking:
In the finance and banking sector, Generative AI proves instrumental in fortifying cybersecurity against emerging threats like phishing and identity theft. By analyzing vast amounts of financial data, it helps in predicting and preventing potential cyber-attacks. Furthermore, it enables robust authentication systems, reinforcing secure transactions and shielding customers from financial fraud.
Transportation and Automotive:
The integration of Generative AI in the transportation and automotive industry significantly contributes to cybersecurity by securing autonomous vehicles and transportation networks. It facilitates real-time threat detection, ensuring safe and uninterrupted operations. By continuously monitoring and analyzing data, it pre-empts potential cyber intrusions, guaranteeing passenger safety and system reliability.
E-commerce and Retail:
In the realm of e-commerce and retail, Generative AI proves invaluable in protecting customer transactions and personal data. It aids in creating secure online platforms, thwarting hacking attempts, and ensuring a seamless shopping experience. Additionally, it assists in fraud detection, swiftly identifying and mitigating any fraudulent activities, safeguarding both businesses and customers.
Government and Public Services:
It enhances incident response, enabling rapid and accurate identification of potential risks. Public services benefit from Generative AI through robust data protection mechanisms. Sensitive citizen data is shielded, ensuring compliance with stringent privacy regulations. The technology’s adaptability aids in countering ever-evolving cyber threats seamlessly. In governmental sectors, Generative AI emerges as a potent ally in cybersecurity endeavors. Its proactive approach significantly strengthens national security infrastructures against cyberattacks. As it continues to evolve, Generative AI promises a safer digital realm for all.
Also Read: Top 5 Career Options in Cybersecurity
Relevance to cybersecurity
- Generative AI plays a pivotal role in bolstering cybersecurity efforts worldwide. Its significance cannot be overstated. As the digital landscape grows ever more complex, so do the threats lurking within it. Cyberattacks have become highly sophisticated, often outpacing traditional security measures. Generative AI empowers cybersecurity professionals to stay ahead of malicious actors by spotting unusual patterns. Its relevance lies in its unique ability to adapt and evolve.
- Traditional cybersecurity systems rely on predefined rules and patterns, leaving them vulnerable to novel attack methods. Generative AI, on the other hand, can analyze vast amounts of data, identifying subtle anomalies that might go unnoticed by human analysts or conventional security tools. This adaptability is crucial in the face of ever-evolving threats.
- Moreover, Generative AI can assist in predictive analysis, helping organizations anticipate potential attacks and vulnerabilities before they are exploited. Its role in threat intelligence is invaluable. Beyond detection and prediction, Generative AI aids in real-time response. It can autonomously neutralize threats as they emerge, reducing response times from minutes to milliseconds. This rapid response is critical in preventing data breaches and minimizing damage. Furthermore, Generative AI enhances the overall resilience of cybersecurity systems.
Generative AI Applications in Cybersecurity
Application | Description |
Malware Detection | Generative AI can analyze patterns in code and generate signatures to detect new and evolving malware strains. |
Phishing Email Detection | It can identify and generate countermeasures against phishing emails by analyzing email content and sender behavior. |
Anomaly Detection | Generative models can establish baselines for network and user behavior, flagging anomalies and potential threats. |
Password Cracking | AI can generate password lists and test against hashed passwords to assess system vulnerabilities. |
Threat Intelligence | AI models can generate reports on emerging threats by analyzing vast amounts of data from various sources. |
Security Policy Creation | It can assist in the creation of security policies by generating recommended configurations based on best practices. |
Adversarial Attack Defense | Generative AI can be used to create robust models to defend against adversarial attacks on AI systems. |
Intrusion Detection | AI can generate alerts when suspicious activities are detected within a network, helping to prevent data breaches. |
Threat detection and prevention
Generative AI revolutionizes cybersecurity by swiftly detecting and preventing a myriad of emerging threats. Traditional methods often struggle to keep pace with the constantly evolving threat landscape. Leveraging advanced algorithms, Generative AI actively analyzes vast datasets to identify potential security breaches.
Its real-time monitoring capabilities allow for proactive threat mitigation, minimizing potential damage and ensuring business continuity. By learning from historical data, Generative AI enhances threat prediction accuracy, enabling organizations to stay one step ahead of potential attacks.
Anomaly detection and pattern recognition
Generative AI’s prowess lies in its ability to detect anomalies and unusual patterns within a network or system. This could be a potential sign of a cyber intrusion. Traditional methods often struggle to discern these subtle deviations. The AI algorithm comprehensively studies normal behavior patterns, enabling it to flag any irregular activities promptly. This facilitates a rapid response, mitigating potential damage and reducing recovery time and costs. Employing Generative AI in anomaly detection augments cybersecurity efforts by providing a deep understanding of complex network behaviors.
Phishing detection and response
Phishing remains a pervasive threat, exploiting human vulnerabilities to gain unauthorized access. Generative AI steps in by efficiently recognizing phishing attempts through sophisticated pattern recognition and behavioral analysis. Utilizing machine learning, Generative AI identifies patterns in phishing emails, URLs, or messages, swiftly alerting users to potential threats. This proactive response protects users from inadvertently divulging sensitive information. Moreover, the AI continually learns from new phishing attempts, evolving its detection techniques to stay ahead of cybercriminals. This iterative improvement strengthens the overall security posture against phishing attacks.
Also Read: How to Start a Cyber Security Career in 2024?
Advantages of Generative AI in Cybersecurity
Enhanced threat intelligence
Traditional methods of threat analysis often struggle to keep pace with the rapid evolution of cyber threats. Generative AI, on the other hand, processes and analyzes vast amounts of data swiftly and accurately. It deciphers patterns and anomalies that could signify a potential breach, providing a proactive stance against cyber threats. This advanced AI can recognize subtle signs of an impending attack. These signs may be too nuanced for human analysts to notice amidst the sheer volume of data. Moreover, Generative AI can simulate various attack scenarios. This capability allows cybersecurity teams to anticipate potential strategies hackers might employ. By understanding these strategies, organizations can fortify their defenses, making it harder for cybercriminals to exploit vulnerabilities.
Automation and real-time monitoring
Generative AI automates repetitive security tasks, liberating human resources for more strategic initiatives. This leads to a proactive approach to threats, with AI constantly monitoring and analyzing for anomalies. The real-time insights provided by Generative AI allow for rapid decision-making and effective incident response. Through automation, it responds to threats swiftly and efficiently, reducing potential damage and minimizing response times. The ability to adapt and learn from new threats makes Generative AI a dynamic tool in cybersecurity.
Scalability and cost-efficiency
Generative AI revolutionizes cybersecurity with its scalable and cost-effective solutions.
In a rapidly evolving digital landscape, scalability is vital to match growing security demands. Generative AI adapts seamlessly, scaling up or down based on the organization’s needs. This scalability ensures that cybersecurity remains robust even during periods of significant expansion. Moreover, it’s a budget-friendly solution, optimizing costs while maintaining high standards of security. By scaling resources judiciously, Generative AI minimizes unnecessary expenses, enhancing cost-efficiency. Businesses can allocate funds more strategically, focusing on other critical aspects while ensuring comprehensive security. In essence, Generative AI not only fortifies cybersecurity but also smartly manages financial resources, a win-win scenario for modern businesses.
Also Read: Blockchain in Construction and Infrastructure Management
Ethical and Privacy Considerations
Consideration | Description |
Respect for Autonomy | Ensuring individuals’ right to control their personal information. |
Data Collection and Storage | Safeguarding data with consent and secure storage. |
Beneficence | Maximizing benefits while minimizing harm in data usage. |
Data Access and Sharing | Regulating data access to prevent misuse. |
Non-Maleficence | Avoiding harm and privacy violations in data handling. |
Transparency | Providing clear information about data usage. |
Justice and Fairness | Ensuring fairness and avoiding discrimination in data processing. |
Anonymization and Pseudonymization | Protecting individual identities in data. |
Accountability | Holding organizations responsible for data handling. |
Consent Mechanisms | Implementing clear and informed consent processes. |
Data privacy and consent
Data privacy is a paramount concern in today’s digital landscape, and Generative AI addresses it effectively. Generative AI emphasizes obtaining clear and informed consent before collecting and processing any personal data. This ensures that individuals have control over how their data is used and offers transparency in data handling. Through Generative AI, organizations can implement robust data anonymization techniques, protecting identities while utilizing valuable data. The ethical use of Generative AI involves continuous monitoring and assessment to prevent misuse and maintain public trust. Respecting data privacy and gaining consent are vital pillars for responsible integration of Generative AI in cybersecurity.
Bias and fairness in AI-powered cybersecurity
Ensuring fairness in AI-powered cybersecurity involves constant vigilance. It’s about identifying and mitigating biases within the AI algorithms, striving for impartiality in threat detection and response. Experts in the field emphasize the need for diverse and inclusive datasets during AI model training.
To maintain trust in AI-powered cybersecurity, transparency is key. Organizations must be transparent about their use of AI in their security infrastructure. This includes disclosing the capabilities and limitations of these systems. Furthermore, accountability mechanisms should be in place. When AI systems make decisions, there must be clear responsibility assigned for those decisions.
Legal implications and regulations of Generative AI in Cyber Security
Legal Implications | Regulations |
Data Privacy | – General Data Protection Regulation (GDPR)
– California Consumer Privacy Act (CCPA) – Health Insurance Portability and Accountability Act (HIPAA) |
Intellectual Property | – Copyright Act
– Trademark Act – Patent Law |
Employment Laws | – Fair Labor Standards Act (FLSA)
– Title VII of the Civil Rights Act – Family and Medical Leave Act (FMLA) |
Environmental Regulations | – Clean Air Act
– Clean Water Act – Environmental Protection Act |
Securities and Exchange | – Securities Act of 1933
– Securities Exchange Act of 1934 – Sarbanes-Oxley Act of 2002 |
Antitrust Laws | – Sherman Act
– Clayton Act – Federal Trade Commission Act |
Challenges and Limitations of Generative AI in Cyber Security
Challenges | Limitations |
1. Technological Advancements | Rapid advancements require constant updating and integration, posing a challenge to keep up with evolving technologies. |
2. Cybersecurity Threats | Persistent and evolving cybersecurity threats pose a significant challenge to data protection and system integrity. |
3. Regulatory Compliance | Compliance with varying international and local regulations can be challenging and resource-intensive. |
4. Data Privacy and Ethics | Balancing the use of data for innovation while maintaining privacy and ethical standards is a persistent challenge. |
5. Scalability | Scaling up operations and infrastructure to meet growing demands without compromising performance and quality is a considerable challenge. |
Overcoming data scarcity and quality issues
- Yet, it’s often a challenge to gather sufficient high-quality data. Generative AI steps in to fill this void. By synthesizing data, it compensates for scarcity and enhances its quality. Generative AI bridges this gap by generating simulated data to augment the limited real-world data.
- This augmentation enriches the dataset, empowering machine learning models to make more accurate predictions. By synthesizing diverse data, Generative AI enhances the robustness and reliability of cybersecurity systems. In essence, it compensates for the lack of extensive, high-quality data that’s crucial for strong defenses.
- Moreover, Generative AI ensures the data used is relevant, avoiding noise that might affect cybersecurity measures. It’s a transformative approach that revolutionizes the efficacy of cybersecurity efforts, especially in data-scarce environments. Generative AI leverages advanced algorithms to create data that mirrors real-world scenarios, optimizing security solutions.
Adversarial attacks and countermeasures
- The digital battlefield is constantly evolving, and cybercriminals are becoming more sophisticated, employing adversarial attacks to outwit conventional security systems. Generative AI understands this cat-and-mouse game. It can adapt and evolve its defenses to counter adversarial tactics.
- Adversarial attacks are cunningly designed to deceive AI systems, exploiting vulnerabilities ingeniously. These attacks manipulate AI algorithms, deceiving them into making erroneous decisions or predictions. Generative AI, however, has demonstrated potential in countering adversarial attacks with improved accuracy.
- By training on diverse and extensive datasets, Generative AI enhances its resilience against adversarial attempts. Advanced Generative AI models are equipped to identify and mitigate adversarial manipulations effectively. They bolster the security infrastructure, offering a robust defense against the ever-evolving adversarial threat landscape.
- Furthermore, continuous research and advancements in Generative AI promise even stronger defenses, staying ahead of adversarial endeavors.
Also Read: Flux- The Future Of Web3 Infrastructure
Explainability and trustworthiness
One significant concern in deploying AI for cybersecurity is the “black box” problem—where decisions made by AI systems are difficult to understand or explain. Generative AI addresses this concern head-on. It not only provides solutions but also explains the reasoning behind its actions. This transparency builds trust among cybersecurity professionals and decision-makers. When you can trust the tools defending your organization, you’re better equipped to face the evolving challenges of the digital age. Generative AI, with its explainable approach, offers a path forward in overcoming this critical limitation.
Conclusion
Generative AI, with its data-crunching prowess, brings enhanced threat intelligence to the table. It deciphers complex patterns, aiding in early threat detection. Automation and real-time monitoring are game-changers. They enable rapid threat identification and free up human resources for strategic decision-making. Scalability and cost-efficiency are no longer mere aspirations. Generative AI offers adaptable solutions that cater to organizations of all sizes, effectively minimizing cybersecurity costs.
Looking ahead, the future of Generative AI in cybersecurity is exceptionally promising. Advancements in machine learning and AI algorithms will further enhance its threat detection capabilities. Generative AI’s ability to evolve and adapt will be vital in countering ever-evolving cyber threats. Collaboration between human experts and AI systems will redefine the cybersecurity landscape, emphasizing synergy. Incorporating ethical considerations will ensure responsible AI utilization, striking a balance between security and ethics. In closing, Generative AI’s role in cybersecurity is poised to grow, shaping a safer digital future.
Frequently Asked Questions
How does Generative AI improve cybersecurity?
- Generative AI enhances cybersecurity by analyzing patterns in data to predict and counter potential cyber threats.
- It aids in incident response, enabling rapid and accurate decision-making during security breaches.
- Generative AI continuously refines its understanding of threats, improving its predictive capabilities.
- It plays a pivotal role in various industries, including healthcare, finance, transportation, e-commerce, and government.
What are the advantages of Generative AI in cybersecurity?
- Generative AI offers enhanced threat intelligence, recognizing subtle signs of impending cyber attacks.
- It automates security tasks, allowing for real-time monitoring and rapid incident response.
- Scalability and cost-efficiency are key advantages, making it adaptable to organizations of all sizes.
- Generative AI compensates for data scarcity and enhances data quality by synthesizing diverse data.
How does Generative AI address ethical and privacy considerations in cybersecurity?
- Generative AI emphasizes obtaining clear and informed consent before collecting and processing personal data.
- It implements robust data anonymization techniques, protecting identities while utilizing data.
- Organizations must maintain transparency in their use of AI in security infrastructure and establish clear accountability mechanisms.
- Generative AI promotes fairness by striving to mitigate biases in AI algorithms.
What are the challenges and limitations of Generative AI in cybersecurity?
- Rapid technological advancements require constant updates and integration, posing a challenge to keep up with evolving technologies.
- Cybersecurity threats are persistent and evolving, posing a significant challenge to data protection and system integrity.
- Compliance with varying international and local regulations can be challenging and resource-intensive.
- Balancing data use for innovation while maintaining privacy and ethical standards remains a persistent challenge.