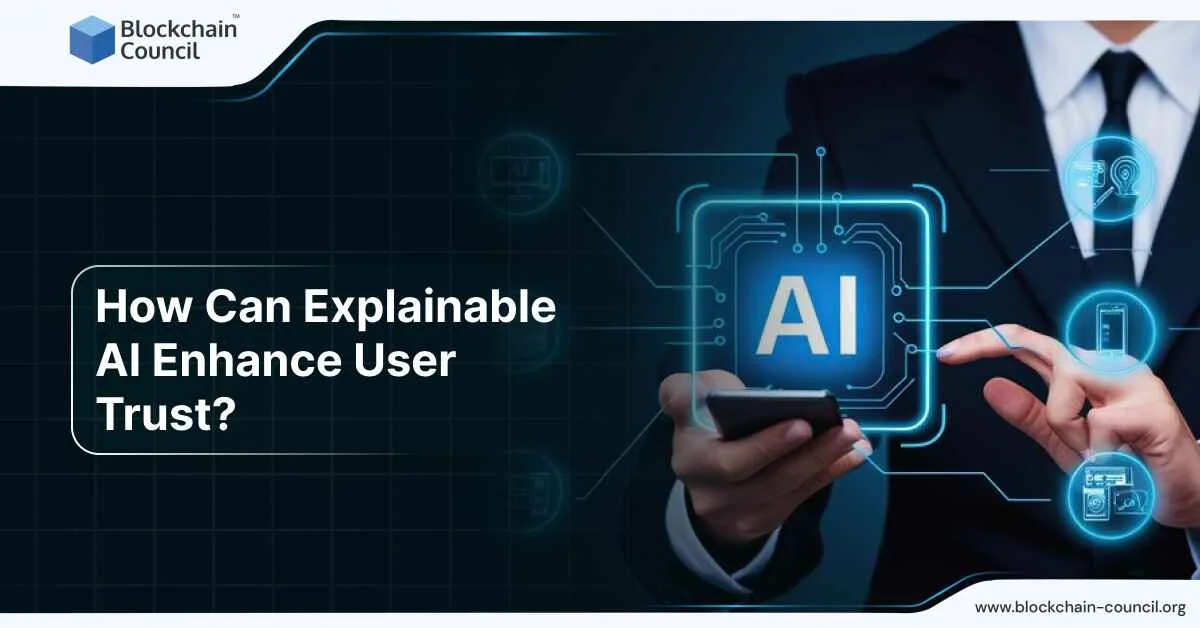
- Blockchain Council
- February 20, 2025
Artificial Intelligence (AI) has shaped industries, influencing areas like healthcare, finance, and autonomous technology. While it plays a major role in progress, AI systems often operate without offering clear reasons for their choices. This lack of transparency raises questions about fairness, accountability, and ethics. Explainable AI (XAI) steps in to provide clarity, ensuring machine-generated decisions make sense to humans.
Breaking Down Explainable AI
XAI includes various techniques that help users understand why AI makes specific decisions. Unlike conventional models, which provide answers without explanation, XAI reveals how different inputs affect final predictions. This increased clarity helps people trust AI while improving interactions between humans and machines.
Why Explainable AI Matters
XAI serves multiple purposes across different fields, such as:
- Improving Trust: Users feel more confident using AI when they understand how it reaches conclusions.
- Legal Compliance: Many industries must follow transparency rules for automated systems. XAI helps companies meet these requirements.
- Ethical Considerations: AI explanations allow users to identify potential biases, ensuring fair outcomes.
- Better Decisions: Understanding how AI arrives at conclusions helps professionals make informed choices.
Methods Used in Explainable AI
Several techniques help interpret AI models effectively.
1. SHAP (SHapley Additive exPlanations)
This method assigns importance values to each feature influencing an AI decision. Based on cooperative game principles, SHAP ensures accuracy and consistency when explaining predictions. In a loan assessment system, for example, SHAP can show how factors like income or credit score affect approval chances.
2. LIME (Local Interpretable Model-Agnostic Explanations)
LIME explains how complex models behave by approximating them with simpler models, focusing on specific predictions. This makes it easier to understand why AI made a particular decision. If an AI system labels an email as spam, LIME highlights words or patterns that led to this classification.
3. Counterfactual Explanations
This technique describes how changing certain inputs could lead to different results. In hiring software, for instance, a counterfactual explanation might reveal that an extra year of experience would have resulted in selection.
4. Saliency Maps
Common in image processing, saliency maps highlight the most influential regions in an image that impacted the model’s decision. This method is widely used in medical imaging to pinpoint areas that led to a diagnosis.
Where Explainable AI is Used
XAI plays a role in various industries, making AI-driven systems more accountable.
1. Healthcare
AI supports doctors by diagnosing diseases and suggesting treatments. However, medical professionals need explanations to trust AI decisions. XAI helps them validate AI recommendations by showing how specific test results or symptoms influenced diagnoses.
2. Finance
Banks and financial institutions use AI for loan approvals, fraud detection, and investment decisions. Transparent AI helps customers understand why applications are rejected and allows businesses to prove that decisions follow fair practices.
3. Autonomous Vehicles
Self-driving cars rely on AI to interpret surroundings and make split-second decisions. XAI can explain why an autonomous vehicle suddenly stopped, detailing detected obstacles or traffic conditions, helping improve safety and trust.
Challenges in Explainable AI
Even though XAI has clear benefits, several obstacles remain:
- Complexity vs. Simplicity: More advanced AI models offer better accuracy, but they are harder to explain. Researchers aim to balance accuracy and clarity.
- Lack of Standardization: There are no universal guidelines to measure AI explainability. Developing industry-wide benchmarks can help improve AI transparency.
- User Awareness: Many people lack basic knowledge of AI workings. Educating users can bridge this gap, making AI explanations more useful.
What’s Next for Explainable AI?
The XAI field continues evolving, with promising advancements ahead.
1. Cross-Disciplinary Research
Experts from psychology, neuroscience, and AI research collaborate to make AI explanations more intuitive, aligning them with human reasoning.
2. Automated Explanation Systems
AI models are now being trained to generate human-like explanations of their decisions. This innovation benefits customer service, legal AI, and financial advising.
3. Hybrid AI Models
Combining rule-based systems with deep learning could enhance both performance and clarity, allowing AI to provide accurate predictions with understandable justifications.
4. Ethical and Regulatory Growth
As AI regulations strengthen worldwide, organizations focus on compliance and transparency. Future AI models will include built-in auditing tools to ensure fair decision-making.
AI Regulations Around the World
Global policymakers are working toward stricter AI transparency laws. Some key developments include:
1. European Union: AI Act
This legislation categorizes AI into risk levels, requiring high-risk AI systems to be explainable, fair, and auditable.
2. United States: AI Bill of Rights & FTC Rules
U.S. regulators push for AI fairness in advertising, credit assessment, and hiring. AI-driven decisions must be transparent to prevent discrimination.
3. China: AI Governance Framework
China enforces strict AI rules, requiring businesses to disclose how AI systems make critical decisions, especially in biometric data and social scoring.
4. Global AI Ethics Standards
Groups like OECD, ISO, and IEEE are working to create universal guidelines for AI transparency and fairness.
Bringing Explainable AI into Business
For companies aiming to integrate XAI, a few key steps can help:
1. Choose the Right Explainability Tools
- Deep learning models: Use SHAP, LIME, or LRP (Layer-Wise Relevance Propagation).
- Decision trees: Feature importance analysis works best.
- Text-processing AI: Attention heatmaps clarify NLP model predictions.
2. Build Explainability from the Start
Instead of treating AI transparency as an afterthought, companies should prioritize it during development by selecting interpretable models.
3. Educate Employees and Users
From engineers to business leaders, everyone should understand AI decisions. Training sessions and clear documentation can improve AI adoption and trust.
4. Monitor AI Models Continuously
Regular audits ensure AI remains unbiased and transparent over time, helping businesses maintain compliance.
Final Thoughts
As AI becomes more embedded in daily life, ensuring transparency isn’t just helpful—it’s necessary. Organizations that prioritize explainability build stronger trust, meet legal standards, and promote ethical AI adoption.
The future will see stronger collaboration between researchers, policymakers, and industries to create AI that is not just accurate but also accountable. Businesses that invest in XAI today will position themselves for long-term success in a world increasingly shaped by AI-driven decisions.