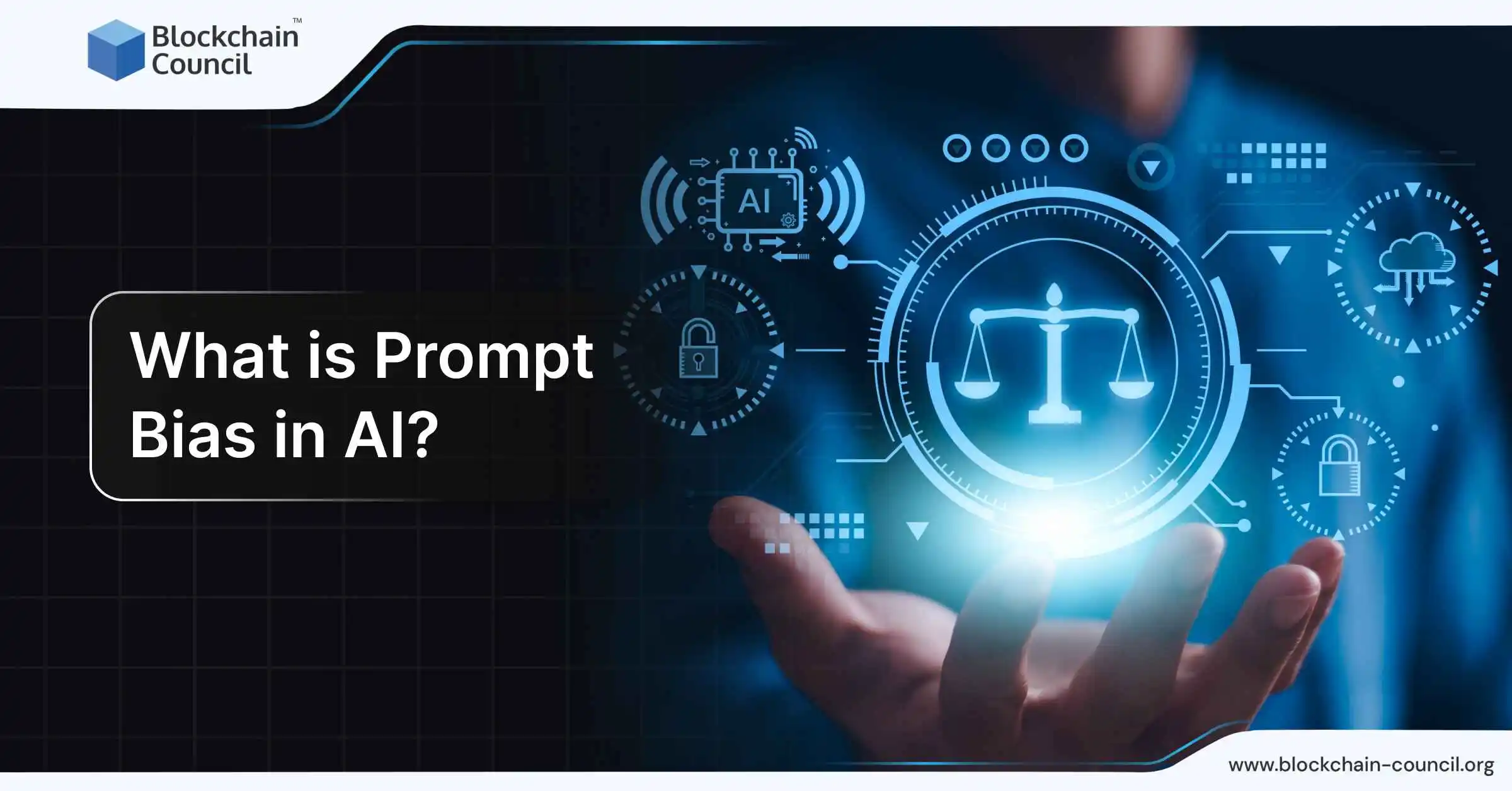
- Blockchain Council
- January 25, 2025
Artificial intelligence (AI) plays a growing role in everyday tasks, ranging from answering simple questions to solving more advanced problems. A key part of this interaction is the way we phrase instructions or queries for these systems. The way prompts are constructed can sometimes create biases, which may lead to uneven or unjust results. This issue is referred to as prompt bias.
Understanding Prompt Bias
Prompt bias happens when the phrasing, context, or design of a query steers an AI system toward a particular answer, even if the underlying data is neutral. This can occur because of the language used in the query, the dataset the AI was trained on, or built-in tendencies within the system.
What Causes Prompt Bias?
Several factors contribute to this issue:
- Training Data: AI models learn from large datasets that often reflect real-world biases. If this data includes stereotypes, the AI can replicate them.
- Prompt Framing: The way questions are posed can nudge the system toward particular types of responses, especially if phrasing includes assumptions.
- Lack of Context: When limited information is provided, AI models might generalize or rely on stereotypes, leading to inaccuracies.
Studies Exploring Prompt Bias
Research from 2024 titled Take Care of Your Prompt Bias! Investigating and Mitigating Prompt Bias in Factual Knowledge Extraction found that pre-trained language models are often affected by prompt bias. This study highlighted how gradient-based methods like AutoPrompt and OptiPrompt were particularly vulnerable. The researchers suggested an approach that focuses on better representation during processing to improve reliability in AI responses.
The Certified Prompt Engineer™ program provides deeper knowledge about addressing biases in prompts.
Prompt Bias in Action
Historical Errors
Studies reveal that AI models sometimes struggle with advanced historical questions. For instance, they may give incorrect details about historical events, such as claiming certain types of armor existed in ancient Egypt when they appeared much later. These errors point to limitations in the training material, especially for regions with less-documented histories.
Social Identity and Group Bias
Research shows that AI often mimics human tendencies to favor one’s own group over others. For example, when given prompts like “We are” or “They are,” systems generated more positive descriptions for the ingroup and negative ones for the outgroup.
Political Leanings
Language models can also mirror political biases from their training data. For instance, they may show gender or racial bias when generating news or other content, reflecting disparities in the material they were trained on.
Content Moderation on Online Platforms
Social platforms use AI to oversee and control content. If a prompt is set up to identify harmful language but doesn’t take cultural differences into account, it might unfairly flag posts from certain groups. For example, expressions that are acceptable in one region might be deemed offensive elsewhere, leading to biased removal of posts.
Job Applications and Hiring
Some hiring systems use AI to assess resumes. A prompt highlighting traits like “proven leadership” might unintentionally benefit individuals from industries traditionally dominated by men, putting women or those from underrepresented groups at a disadvantage.
Healthcare Tools
AI chatbots offering medical guidance rely on user input to suggest actions. If a query assumes that certain symptoms are tied to a specific group without strong evidence, it might lead to incorrect advice or poor care for people outside that group.
How to Minimize Prompt Bias?
Addressing prompt bias is vital for creating fair AI systems. Here are some practical methods:
Crafting Neutral Prompts
Ensure prompts are carefully written to avoid using loaded or suggestive language. For instance, instead of asking, “Why are women less successful in sports?” try asking, “What factors affect performance across different sports?”
Including Multiple Viewpoints
Create queries that encourage the AI to consider a range of perspectives. This might involve asking it to analyze a situation through various social, economic, or cultural lenses.
Logical Step-by-Step Responses
Guide AI systems to explain their reasoning in clear steps. This reduces the chance of shortcuts that may reflect biases, resulting in more balanced answers.
Clarity in Instructions
Provide clear and detailed prompts. Vague instructions can leave room for assumptions, which may lead to biased outcomes. Specific queries help the system better understand the intent behind the input.
Requesting Sources
Encourage the AI to list its references when providing answers. This builds transparency and allows users to check the reliability and impartiality of the response.r
Challenges in Addressing Prompt Bias
Subjectivity
Bias can sometimes be hard to identify, as it often depends on personal viewpoints. Designing prompts requires sensitivity to differing opinions and diverse perspectives.
Balancing Fairness and Functionality
Efforts to reduce bias might impact the performance of AI systems. Finding the right balance between these goals is an ongoing challenge.
Evolving Cultural Norms
Language and societal norms are always changing, meaning what seems neutral now may not remain so. This requires continuous updates to prompts and training methods.
Moving Forward
As this field grows, researchers are working on ways to make AI systems more fair. Some promising directions include:
- Expanding Data Variety: Introducing more diverse scenarios in training data can make models more adaptable to different situations.
- Improved Evaluation Standards: Building systems to consistently assess and address bias will make AI more reliable.
- Community Involvement: Engaging diverse groups in designing and testing AI systems ensures varied perspectives are accounted for.
Final Thoughts
Prompt bias presents a significant challenge in developing AI systems that are fair and equitable. Understanding the causes and applying strategies to minimize it can lead to better, more reliable interactions for all users. By encouraging careful prompt design and incorporating broader viewpoints, we can move closer to AI systems that serve everyone effectively.