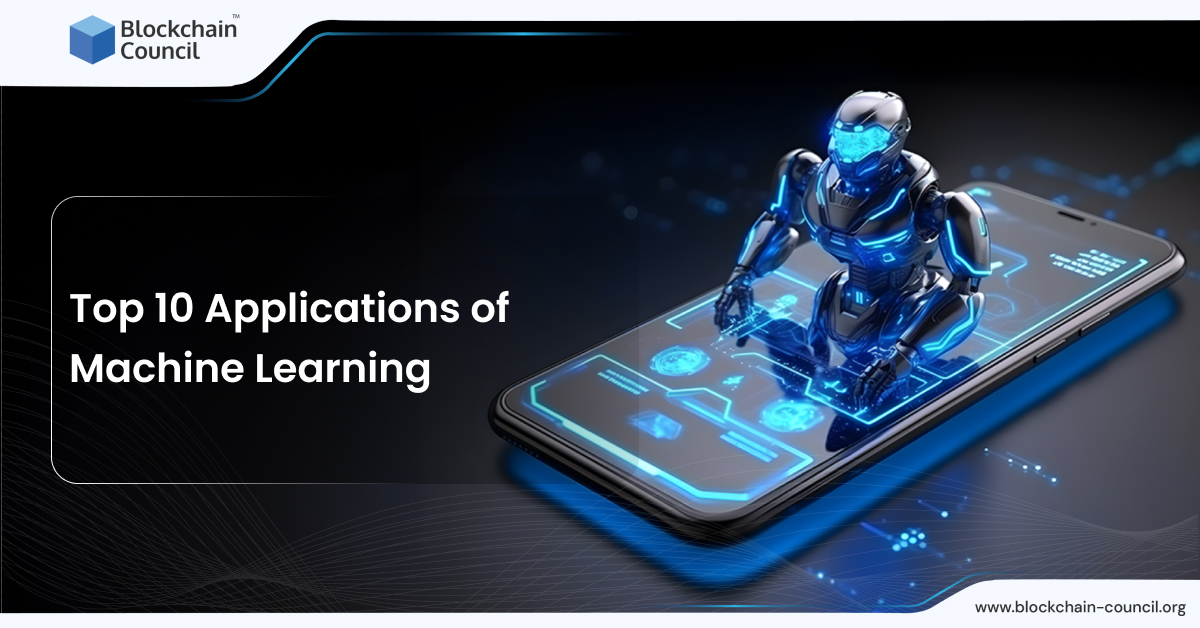
- Blockchain Council
- February 06, 2024
Summary
- Machine Learning (ML) is shaping various industries, enhancing customer experiences, and revolutionizing healthcare with personalized treatment plans and early disease prevention.
- The article discusses the top 10 machine learning applications in 2024.
- In manufacturing, ML enables ‘Smart Manufacturing’ with predictive maintenance, optimized supply chains, and collaborative robotics for efficient and safe environments.
- The financial sector sees ML’s impact on fraud detection, risk management, and personalized financial services, transforming customer experiences in banking and insurance.
- Retail experiences are enhanced through ML with personalized product recommendations, virtual fitting rooms, and cybersecurity to protect online shoppers.
- ML is at the forefront of autonomous vehicles, reducing traffic accidents and transforming public transportation with self-driving buses and trains.
- ML plays a crucial role in environmental protection, predicting weather patterns, optimizing renewable energy sources, and promoting sustainability in agriculture.
- Education undergoes a revolution with ML providing personalized learning experiences, language practice applications, and insights for educators to adapt teaching methods.
- Smart cities benefit from ML in efficient city planning, optimizing traffic flow, managing energy usage, and predicting disease outbreaks for faster responses.
- Entertainment and media witness changes with ML analyzing viewer preferences, assisting in creative processes in film and music, and optimizing training in sports.
- ML contributes significantly to space exploration and astronomy, analyzing data from telescopes, enhancing space mission efficiency, and aiding the search for extraterrestrial life.
- ML is a paradigm shift that redefines how we live, work, and interact, serving as the cornerstone of a more intelligent and personalized future in 2024.
As we step into 2024, Machine Learning (ML) continues to be a pivotal force in driving innovation across various industries. From enhancing customer experiences to revolutionizing healthcare, ML’s footprint is unmistakable. This powerful branch of artificial intelligence has ceased to be a mere buzzword, transforming into a cornerstone of innovation across diverse sectors. Approximately 48% of businesses globally utilize machine learning. And the market size of machine learning is expected to reach $528.10 billion by 2030.
From revolutionizing healthcare with personalized treatments to driving efficiency in smart manufacturing, the applications of machine learning are not just expanding; they are deepening in their impact and sophistication.
This article delves into the top 10 applications of machine learning in 2024, exploring how this technology is not just shaping industries but also redefining our daily lives. So, let’s get started!
10 Best Machine Learning (ML) Applications
1. Personalized Healthcare
Healthcare is undergoing a transformation, thanks to machine learning. In 2024, personalized healthcare is not a luxury but a norm. ML algorithms analyze patient data to provide customized treatment plans, predicting health risks and preventing diseases before they manifest. Wearables and health apps, empowered by ML, constantly monitor vital stats, offering real-time insights to both patients and healthcare providers.
Beyond diagnostics, ML is also transforming therapeutic approaches. Algorithms are being developed to tailor drug dosages to individual genetic profiles, minimizing side effects and maximizing efficacy. In mental health, ML tools analyze speech and behavioral patterns to assist in early diagnosis and personalized treatment plans.
Key Developments
- Mhealth and Telehealth: Utilizing AI for remote healthcare services, transforming delivery methods.
- AI-driven EHS Solutions: Enhancing workplace safety and environmental compliance in healthcare settings.
- Electronic Health Records (EHR): Revolutionized by AI for real-time comprehensive patient data access.
- Medical Technology Advancements: AI is integral in developing robotic surgeries and smart prosthetics.
- AI Investment in Healthcare: Significant funding is being directed towards AI healthcare startups, promising innovative solution
2. Smart Manufacturing
The manufacturing sector is leveraging ML to usher in an era of ‘Smart Manufacturing.’ Predictive maintenance, powered by machine learning, predicts equipment failures before they occur, minimizing downtime and extending machinery life. Furthermore, ML algorithms optimize supply chains, forecast demands, and enhance quality control, leading to efficient and cost-effective production.
Smart Manufacturing doesn’t stop at predictive maintenance and supply chain optimization. Machine learning is also being utilized in enhancing worker safety through the analysis of workplace data to predict and prevent accidents. Additionally, ML-driven robotics are increasingly collaborating with human workers, leading to more efficient and safer manufacturing environments.
Key Developments
- Remote and Off-site Operations: Adoption of cloud-based solutions for remote monitoring and virtual maintenance, integrating IIoT and digital twin technology.
- Collaborative Robots (Cobots): Working alongside human workers for efficiency and safety.
- Machine Health and Condition Monitoring: Widespread adoption of sensors for real-time machine monitoring.
- Predictive Maintenance and Efficiency: Using sensors for proactive maintenance strategies.
- Predictive Operations at Macro Level: Leveraging AI and big data for global scenario analysis and preparedness.
3. Advanced Financial Services
Machine learning is revolutionizing the financial sector by introducing sophisticated algorithms for fraud detection, risk management, and personalized financial services. ML algorithms analyze vast amounts of transaction data to identify fraudulent activities in real-time. Additionally, they play a crucial role in algorithmic trading, using predictive analytics to make informed investment decisions.
In banking, ML algorithms are enhancing customer service through chatbots and personalized banking experiences, offering financial advice based on individual spending habits and financial goals. In the insurance sector, ML is being used for personalized policy pricing, risk assessment, and processing claims more efficiently.
Key Developments
- Digital Technologies Adoption: Financial companies integrating third-party tools for better data analysis and reporting.
- Growth of Alternative Lending Platforms: Increase in non-traditional lending to cater to diverse customer needs.
- Focus on Customer Experience: Banks and insurance companies prioritizing personalized services.
- Financial Inclusion and Accessibility: Digital trends leading to broader access to financial services and loans.
- Technological Innovation and Big Data Impact: Improved customer service and development of innovative financial services through technology like AI-based speech analytics
4. Enhanced Retail Experiences
In the retail sector, machine learning is reshaping the shopping experience. From personalized product recommendations based on shopping behavior to optimizing inventory management, ML’s applications are vast. Virtual fitting rooms and AI-driven customer service bots are becoming commonplace, offering customers a seamless and personalized shopping journey.
The retail sector is also seeing advancements in supply chain management through ML, with algorithms predicting stock levels, optimizing logistics, and reducing waste. In the age of online shopping, ML-driven cybersecurity is becoming increasingly important to protect consumers from fraud.
Key Developments
- Hyper-personalization: Delivering personalized experiences across different platforms and physical stores.
- Automation in Retail: Freeing up employee time for creative and high-value tasks.
- Data-Driven Customer Universe Expansion: New data approaches like data clean rooms, partnerships, and retail media networks for targeted advertising and insights.
- Elevated Content and Personalization Efforts: Extending customer experience to new channels like shoppable TV, livestreaming, and social commerce
5. Autonomous Vehicles
Perhaps the most exciting application of ML in 2024 is in the development of autonomous vehicles. Self-driving cars, equipped with ML algorithms, process data from sensors and cameras to navigate safely. This technology promises to reduce traffic accidents, ease congestion, and revolutionize transportation.
The application of ML in autonomous vehicles extends to public transportation, with the development of self-driving buses and trains. These vehicles are equipped with advanced ML algorithms that not only navigate but also constantly learn from their environment, improving safety and efficiency over time.
Key Developments
- Full Autonomy and Mainstream Adoption: Efforts towards achieving Level 5 autonomy and broader adoption of autonomous vehicles.
- Expansion in Self-Driving Public Transportation: Development of self-driving buses, trains, and other forms of public transport.
- Integration with Smart City Infrastructure: Autonomous vehicles being integrated with smart city technologies for optimized traffic management and urban planning.
- Advanced Safety and Navigation Systems: Utilizing machine learning for improved safety features and more accurate navigation in autonomous vehicles.
- Commercial and Industrial Applications: Expansion of autonomous vehicle technology into areas like freight, agriculture, and construction.
6. Environmental Protection and Sustainability
Machine learning is playing a crucial role in combating climate change and promoting sustainability. By analyzing environmental data, ML algorithms can predict weather patterns, assess the impact of climate change, and optimize renewable energy sources. In agriculture, ML is being used to predict crop yields, optimize irrigation and reduce pesticide usage, contributing to sustainable farming practices.
Machine learning’s impact on environmental protection extends to ocean conservation, where algorithms analyze marine data to track pollution levels and the health of marine ecosystems. In urban settings, ML is used to optimize public transportation systems, reducing carbon emissions and improving air quality.
Key Developments
- AI in Climate Change Prediction and Mitigation: Leveraging AI for accurate climate modeling and developing strategies for climate change mitigation.
- Biodiversity Monitoring and Conservation: Using AI for tracking wildlife and ecosystem health, and combating illegal activities like poaching.
- Sustainable Resource Management: AI applications in optimizing the use of natural resources and promoting sustainable practices in various industries.
- Pollution Monitoring and Control: Implementing AI-driven solutions for monitoring air and water quality and managing pollution.
- Energy Efficiency and Renewable Energy Optimization: AI algorithms optimizing renewable energy sources and enhancing energy efficiency in various sectors.
7. Education and Personalized Learning
The education sector is being revolutionized by ML through personalized learning experiences. By analyzing student performance data, ML algorithms can tailor educational content to individual learning styles and needs, making education more effective and accessible. In higher education and professional training, ML is enabling the development of intelligent tutoring systems that provide personalized guidance and feedback.
In the realm of language learning, ML-powered applications offer real-time translation and language practice, making language learning more accessible and effective. For educators, ML provides valuable insights into student engagement and learning patterns, enabling them to adapt teaching methods for better outcomes.
Key Developments
- Social and Emotional Wellbeing: Focus on mental health programs and technologies for student and teacher wellbeing.
- Personalized and Self-Led Learning: Individualized learning opportunities and active learning spaces.
- Game-Based Learning and Esports: Incorporating gamified learning and building esports teams in schools.
- Microlearning and Nano-Learning: Bite-sized learning techniques using short, digestible tutorials or mini-lessons.
- AR, VR, and AI in Education: More prevalent use of augmented reality, virtual reality, and AI as educational tools.
8. Smart Cities and Urban Planning
Machine learning is at the forefront of developing smart cities. By analyzing data from various sources like traffic patterns, utility usage, and public safety incidents, ML algorithms are helping in efficient city planning and management. This includes optimizing traffic flow, improving energy efficiency, and enhancing public safety through predictive policing and emergency response.
Smart city initiatives also include ML-driven energy management systems in buildings, optimizing energy usage and reducing costs. In terms of public health, ML algorithms help in monitoring and predicting disease outbreaks in urban areas, enabling faster and more targeted responses.
Key Developments
- Sustainable Energy Management: Focus on renewable energy sources, energy-efficient buildings, and smart grids.
- Smart Mobility: Intelligent traffic management, eco-friendly transportation, autonomous vehicles, and car-sharing.
- Tech Solutions for Digital Citizens: Remote healthcare, interactive education, and entertainment.
- Blockchain for Safety and Security: Enhancing safety and security measures for residents.
- Advancements in Robotics, Extended Reality, and Drones: Empowering urban planning, agriculture, and waste management.
9. Entertainment and Media
In the world of entertainment and media, ML is changing the way content is created, distributed, and consumed. Algorithms are being used to analyze viewer preferences and create personalized content recommendations. In the film and music industries, ML is assisting in the creative process, from script writing to composing music.
In sports, ML is used for performance analysis, providing coaches and athletes with insights to optimize training and strategies. In journalism, algorithms assist in analyzing trends and automating parts of the news reporting process, allowing journalists to focus on in-depth reporting.
Key Developments
- Hyper-Personalization: Focusing on understanding and anticipating customer needs for tailored media experiences.
- Data Utilization for Engagement and Retention: Using data analytics to enhance customer retention and value.
- Generative AI for Content Creation: Using AI to create more and better content, and reaching the right customers.
- Emergence of New Social Platforms: Platforms like TikTok and BeReal becoming central to media and brand engagement, especially for Gen Z.
- Creativity and Automation in Content Management: Streamlining content lifecycles and employing automation to enhance creative processes in media production.
10. Space Exploration and Astronomy
Machine learning is also playing a pivotal role in space exploration and astronomy. ML algorithms are analyzing data from telescopes and space missions to discover new celestial bodies and understand cosmic phenomena. In space missions, ML is used for autonomous navigation and data analysis, significantly enhancing the efficiency and success rate of these missions.
In the field of astrobiology, ML helps in analyzing data from extraterrestrial environments, aiding in the search for signs of life beyond Earth. The technology is also instrumental in developing autonomous systems for interstellar probes, allowing them to navigate and conduct research in deep space autonomously.
Key Developments
- Deep Space Navigation and Automation: Advanced ML algorithms are being used for autonomous navigation and operation of spacecraft in deep space missions.
- Exoplanet Discovery and Analysis: Machine learning aids in analyzing vast amounts of data from telescopes to identify and study exoplanets in distant solar systems.
- Astronomical Data Processing: ML algorithms process and interpret massive datasets from astronomical observations, helping in the discovery of new celestial bodies and phenomena.
- Predictive Maintenance for Spacecraft: Using ML for predictive maintenance of spacecraft systems, ensuring longer missions and reducing the risks of failures.
- Astrobiology and Extraterrestrial Life Search: ML tools analyze data from extraterrestrial environments, aiding in the search for signs of life and understanding alien ecosystems.
Conclusion
The exploration of machine learning’s top applications in 2024 reveals a world where technology and human experience converge in extraordinary ways. Machine learning is no longer a distant future concept; it has become an integral part of the fabric of modern life. The advancements in healthcare, manufacturing, finance, retail, and autonomous vehicles underscore a larger narrative of technological empowerment and innovation. 73% of business leaders believe that machine learning will improve productivity, and 85% of executives think it gives their company a competitive advantage. What’s more?
A recent survey stated that 80% of individuals believe that machine learning tends to increase business revenue rather than decrease expenses. As we look beyond 2024, the potential of machine learning is limitless, promising to unlock new horizons in various fields. And with the advancements in this field, career opportunities will increase as well. There is an expected 22% annual increase in the employment of machine learning engineers from 2023 to 2030. However, companies face challenges in finding skilled staff, with 56% reporting this as a major hurdle in implementing AI and ML. So, how to grab a high paying job in this field? Consider being certified by a reputed organization by the Blockchain Council and ensure your six figure salary at top-notch companies.
However, as we embrace these advancements, we must also navigate the ethical considerations and societal impacts they bring. The journey of integrating machine learning into our world is not just about technological achievement; it’s about shaping a future that is more efficient, more informed, and ultimately, more human. The story of machine learning is still being written, and we all have a role in ensuring it leads to a future that benefits everyone.
Frequently Asked Questions
What is the application of machine learning?
- ML is applied in personalized healthcare, analyzing patient data for tailored treatment plans and predicting health risks.
- In smart manufacturing, it enables predictive maintenance, optimizes supply chains, and collaborates with robotics for efficient operations.
- ML revolutionizes financial services by detecting fraud, managing risks, and providing personalized financial solutions.
- Retail experiences are enhanced through ML with personalized product recommendations, virtual fitting rooms, and cybersecurity.
- ML is used to improve customer experiences, personalize healthcare, and optimize processes in various industries.
- It aids in predicting and preventing issues, reducing downtime, and extending the life of equipment in manufacturing.
- ML in finance detects fraudulent activities, manages risks, and provides personalized financial services.
- In retail, it optimizes inventory management, provides personalized recommendations, and enhances cybersecurity.
Who uses machine learning in daily life?
- Individuals benefit from ML in personalized healthcare through wearables and health apps for real-time monitoring.
- Consumers experience ML in retail with personalized product recommendations and AI-driven customer service bots.
- ML is used by banking customers through chatbots and personalized banking experiences.
- Public transportation users encounter ML in autonomous vehicles, reducing traffic accidents and congestion.
Where are machine learning used examples?
- ML is used in personalized healthcare for predicting health risks and providing customized treatment plans.
- In manufacturing, ML enables predictive maintenance and collaborates with robotics for efficiency.
- Financial services apply ML for fraud detection, risk management, and personalized customer experiences.
- Retail utilizes ML for personalized product recommendations, virtual fitting rooms, and cybersecurity.
- ML is used in industry for predictive maintenance, optimizing supply chains, and enhancing quality control in manufacturing.
- In finance, ML is applied for fraud detection, risk management, and personalized financial services.
- Retail industry utilizes ML for personalized experiences, optimized inventory management, and cybersecurity.
- ML is employed in autonomous vehicles, reducing accidents, easing congestion, and transforming transportation.