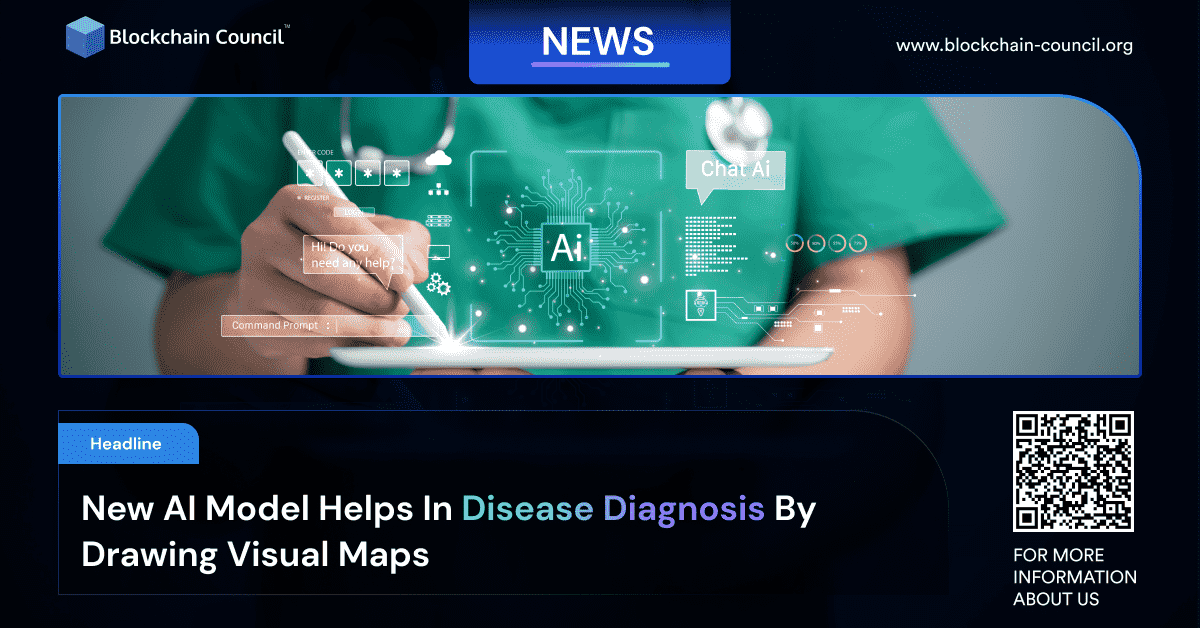
- Blockchain Council
- September 12, 2024
Researchers at the Beckman Institute have pioneered a groundbreaking artificial intelligence (AI) model designed to revolutionize disease diagnosis by providing transparent visual maps alongside each diagnosis. This innovative tool promises to empower medical professionals, enhance patient understanding, and transform the landscape of healthcare.
The AI model, aptly dubbed a medical diagnostics expert, doctor’s assistant, and even a cartographer, has been meticulously developed to accurately pinpoint tumors and diseases in medical images. What sets this model apart is its ability to articulate the reasoning behind each diagnosis through intuitive visual maps. This transparency not only facilitates doctors in comprehending the AI’s decision-making process but also enables them to effectively communicate and reassure patients about their results.
Lead author Sourya Sengupta, a graduate research assistant at the Beckman Institute, emphasizes the model’s pivotal role in early disease detection, likening it to an “X on a map.” By shedding light on how decisions are made, the model aims to streamline the diagnostic process, alleviating the burden on both medical professionals and patients.
The research, published in IEEE Transactions on Medical Imaging, marks a significant milestone in the realm of AI-driven healthcare. Since its inception in the 1950s, artificial intelligence has evolved exponentially, with machine learning (ML) emerging as a cornerstone methodology. ML serves as a training ground for AI systems, equipping them with the capacity to adapt, analyze, and problem-solve akin to human cognition.
Deep learning, a more advanced iteration of ML, harnesses the prowess of deep neural networks to tackle complex tasks with unparalleled precision. However, these intricate networks often grapple with the “black box problem,” wherein they struggle to elucidate their decision-making rationale.
Sengupta draws an insightful analogy, likening deep neural networks to overzealous toddlers who may get things right but lack the ability to articulate their reasoning. This inherent opacity poses significant challenges, particularly in critical domains such as medical image interpretation.
Addressing this challenge head-on, Sengupta and his team introduce a groundbreaking approach that transcends the conventional black box paradigm. Their novel AI model not only discerns anomalies within medical images but also elucidates its decision-making process through visual maps, or equivalency maps (E-maps).
These E-maps serve as dynamic representations of the original images, assigning numerical values to different regions based on their diagnostic significance. By scrutinizing these maps, doctors can discern the underlying rationale behind the AI’s diagnosis, fostering transparency and trust in the patient-provider relationship.
The efficacy of this model was rigorously evaluated across three distinct disease diagnosis tasks, encompassing over 20,000 medical images. Impressively, the model exhibited comparable accuracy rates to existing black-box AI systems, thus affirming its viability as a transformative diagnostic tool.
Principal investigator Mark Anastasio underscores the profound societal impact of this innovation, emphasizing its potential to not only enhance disease diagnoses but also foster trust and transparency within the healthcare ecosystem.
Indeed, the advent of this AI-driven paradigm promises to usher in a new era of healthcare characterized by transparency, efficacy, and patient-centricity. As medical professionals and AI converge to tackle the complexities of disease diagnosis, the future of healthcare looks brighter than ever before.